- The AI Finance Frontier
- Posts
- Algorithmic trading based on a risk-return reinforcement learning
Algorithmic trading based on a risk-return reinforcement learning
Deep Reinforcement Learning Application to Algorithmic Trading
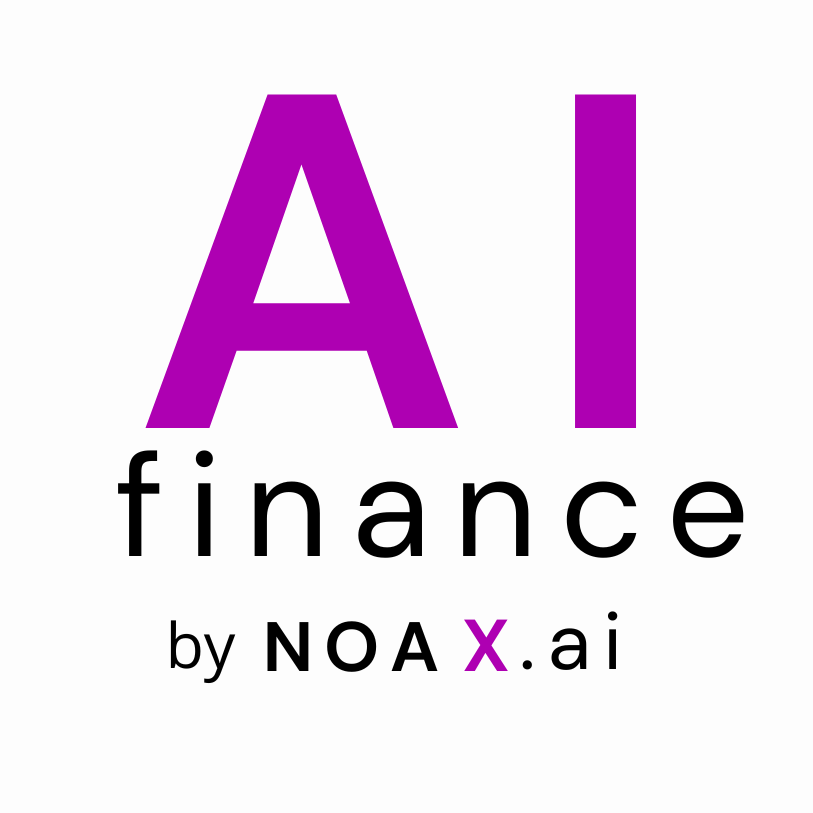
Hi! Here's Iván with this week's exciting newsletter, brimming with insights and discoveries on building robust investment strategies and risk models using Machine Learning.
In this edition, I am presenting the following sections:
🕹️ AI-Finance Insights: I summarize three must-read academic papers that mix cutting-edge ML/DL with Quant Finance:
Algorithmic trading based on a risk-return reinforcement learning algorithm
Deep Learning for Enhanced Index Tracking
Stock market index prediction using deep Transformer model
💊 AI Essentials: The section on top AI & Quant Finance learning resources: Today, I'm introducing a free newsletter that keeps you updated on the hottest AI discoveries and news in less than 5 minutes.
🥐 Quant Finance Insights: In this edition, I provide a method to combine short-term reversal and trading volume to enhance any short-term momentum-based strategy.
Today’s Sponsor
Are you ready to take your investing game to the next level?
We empower investors like you with a daily newsletter which includes the insights needed to stay informed and make profitable decisions in the stock market.
Join Millions Who Trust The Early Bird
Discover daily recommendations
Unlock hidden opportunities
Make informed investment decisions
By receiving our #1 stock tip delivered straight to your inbox every day at 6:59 am—completely FREE.
Seize the Opportunity:
Join the ranks of millions of investors who trust The Early Bird. Sign up now to receive today's #1 Trade of the Day and unlock a world of exclusive benefits.
Your success in the market is just one click away!
AI-Finance Insights
“Algorithmic trading based on a risk-return reinforcement learning algorithm”

Another piece of research applies Reinforcement Learning to trading strategies from a different approach. As always, take it with a grain of salt and use it to stimulate your own trading ideas based on it.
Here, I summarize the paper in a nutshell: 👇
➡ This paper talks about a new way to figure out the best mix of investments using a smart computer program. It tries to make good trading choices based on past sales and how well financial things like stocks are doing.
➡ It uses a special setup where one part of the program (the critic) learns about how returns add up over time, and another part (the actor) picks the best mix to make the most money while keeping risk in mind.
➡ The method also includes a simple way to sell assets you don't own yet (short selling) and uses a fast training method called Ape-x to teach the computer program quickly.
➡ To show it works, they tested it on two different sets of investments and found it did better than other common strategies. This shows this new approach could be really useful for managing investments.
“Deep Learning for Enhanced Index Tracking”

How can DL/ML be used to improve the index-tracking problem? In this paper, the authors explore this application for a stock index by trading some of its constituents using DL.
Here, I summarize the paper in a nutshell: 👇
➡ The paper introduces a groundbreaking deep learning approach to solving the enhanced index tracking problem, aiming to beat a financial index while managing the tracking error effectively.
➡ It proposes a dynamic trading strategy, developed from a neural network that processes various feature types, including index and stock behaviors, short-term characteristics, and current allocations, through four distinct blocks in its architecture.
➡ The outputs from these blocks are merged to adjust the portfolio allocation, aiming for optimal investment combinations.
➡ Tested on the S&P 500 index using real market data, the model's out-of-sample performance highlights the significance of these diverse features and showcases its capability to achieve notable excess returns with effective control over tracking error, downside risk, and transaction costs.
“Stock market index prediction using deep Transformer model”

This work presents another empirical example of the potential applications of transformers for financial time series prediction.
As always, take it merely as a source of ideas to start with.
Here, I summarize the paper in a 5 takeways: 👇
➡ The authors use deep learning for predicting the movements of financial markets. The paper highlights the superior capabilities of deep learning models, such as convolutional and recurrent neural networks, in understanding the complex, non-linear patterns of the stock market.
➡ These advanced models significantly enhance the accuracy of stock market index predictions. The core of the study is the application of the Transformer framework, a tool originally developed for natural language processing tasks, now innovatively adapted for time series forecasting in the financial domain.
➡ The Transformer's unique encoder-decoder architecture and its multi-head attention mechanism enable a more nuanced analysis of stock market trends. This allows for a deeper insight into the underlying dynamics that govern market movements.
➡ The paper's validity is tested through extensive back-testing on several major global stock indices, including the CSI 300, S&P 500, Hang Seng Index, and Nikkei 225. The results of these experiments are compelling, showing that the Transformer model significantly outperforms traditional methods in predicting market trends.
➡ This leads to the potential for higher profits for investors, marking a significant step forward in the field of algorithmic trading. The study's findings open up new avenues for developing more sophisticated and profitable trading strategies, leveraging the power of deep learning technologies.
AI-Essentials
How do you stay up-to-date with the insane pace of AI? Join The Rundown – the world’s fastest-growing AI newsletter with over 500,000+ readers learning how to become more productive using AI every morning.
1. Our team spends all day researching and talking with industry experts.
2. We send you daily updates on the latest AI news, tools, and tutorials.
3. You learn how to become 2x more productive by leveraging AI.
Quant Finance Insights
“Short-term Momentum: Reversal vs Trading Volume”

Short-term reversal in momentum-related strategies is well-known. However, the short-term reversal is concentrated only on those stocks with low trading volumes.
Combining both facts substantially increases the effectiveness of any short-term reversal strategy.
Here, I summarize the paper in less than 2 min: 👇
➡ The study unveils a notable pattern in both U.S. and international stock markets, demonstrating how stocks' performance can be predicted by looking at the previous month's returns and trading volumes.
➡ It finds that stocks with low trading volumes tend to reverse their short-term trends, while those with high volumes continue to follow the momentum established in the short term. This momentum is found to be as lucrative and enduring as the traditional price momentum.
➡ Notably, this momentum effect persists even after accounting for transaction costs and is most pronounced among stocks that are large, liquid, and widely followed.
➡ The findings challenge traditional models based on strict rational market behavior, suggesting instead that they may be better explained by some traders not fully appreciating the informational value of stock prices.
If you're enjoying our newsletter and want to support us, please recommend it to anyone you know who's interested in AI and Finance. Your referrals are the biggest compliment and help us grow! 🌟🤖💼