- The AI Finance Frontier
- Posts
- Building Cross-Sectional Systematic Strategies By Learning to Rank
Building Cross-Sectional Systematic Strategies By Learning to Rank
Learning-to-rank algorithms can significantly improve the risk-adjusted returns.
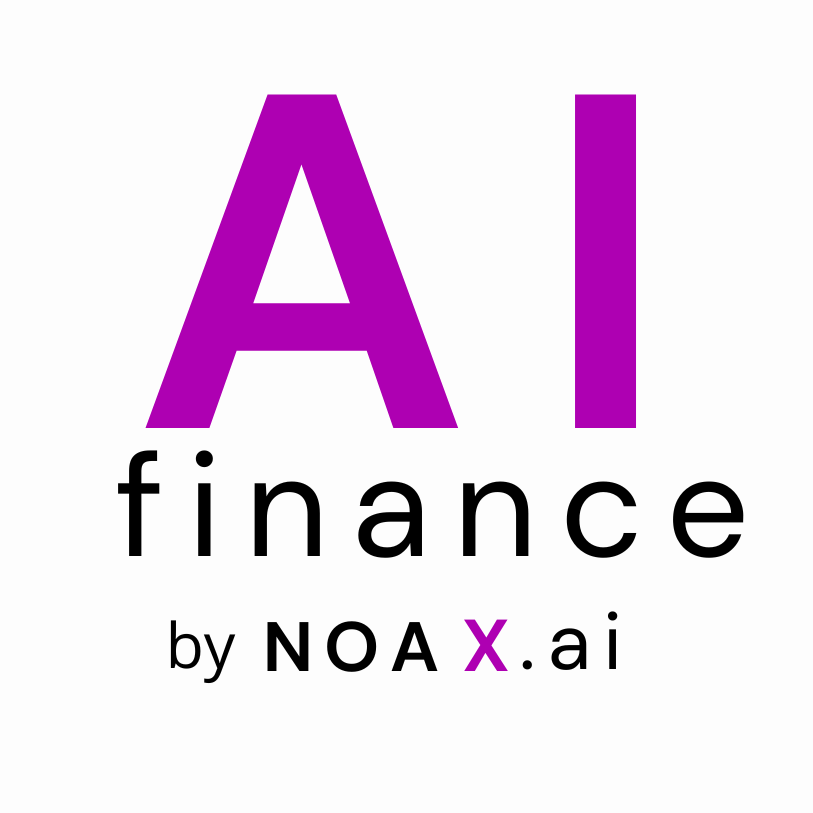
Hi! Here's Iván with this week's exciting newsletter, brimming with insights and discoveries on building robust investment strategies and risk models using Machine Learning.
In this edition, I am presenting the following sections:
🕹️ AI-Finance Insights: I summarize three must-read academic papers that mix cutting-edge ML/DL with Quant Finance:
Building Cross-Sectional Systematic Strategies By Learning to Rank
Comparing Deep Learning Models for the Task of Volatility Prediction Using Multivariate Datas
Return Predictability: Accounting versus Market Information
💊 AI Essentials: The section on top AI & Quant Finance learning resources: Today, I am sharing a "long tutorial" on autoencoders that, in my view, is one of the most comprehensive and detailed tutorials on the topic available for free on the internet.
🥐 Quant Finance Insights: In this edition, I recommend a paper on detecting and predicting market regimes using statistical jump models.
Today’s Sponsor
Ready to elevate your investing journey to new heights?
Unlock the power of informed decision-making with our daily newsletter, tailored to empower investors like you. The Early Bird delivers crucial insights that keep you ahead in the stock market, ensuring you make profitable decisions.
Why Join The Early Bird?
Join millions who trust us
Discover daily recommendations
Unlock hidden opportunities
Make informed investment decisions
AI-Finance Insights
“Building Cross-Sectional Systematic Strategies By Learning to Rank”

In the paper, the authors (from Oxford-Man Institute of Quantitative Finance, University of Oxford) present a method for enhancing stock ranking in cross-sectional investment strategies.
They demonstrate how utilizing "learning-to-rank" algorithms can significantly improve the risk-adjusted returns of these strategies.
Here are my 4 main takeaways from the paper: 👇
➡ The study focuses on improving how assets are ranked before creating an investment portfolio. It challenges old ranking methods with a new approach using learning-to-rank algorithms, which are more effective.
➡ Learning-to-rank algorithms, which consider relationships between assets, are shown to more accurately rank assets, especially when considering cross-sectional momentum—a strategy that bets on continuing trends.
➡ By testing on major US stock indexes, the study finds that using these advanced algorithms can triple the performance of investment strategies compared to traditional methods.
➡ This research is valuable for portfolio managers. It highlights how learning-to-rank algorithms can lead to better investment decisions, offering a significant upgrade over older methods.
“Comparing Deep Learning Models for the Task of Volatility Prediction Using Multivariate Data”

The paper evaluates different deep learning models for forecasting financial volatility with multivariate data.
It transitions from basic architectures to advanced, complex ones, checking their efficacy against naïve forecasts and classical GARCH models for vol of assets like the S&P 500, NASDAQ 100, gold, silver, and oil.
The Temporal Fusion Transformer and Temporal Convolutional Network notably outperform traditional methods, with their superiority confirmed through repeated experiments and statistical analysis, underscoring their potential practical benefits.
Here are my 4 main takeaways from the study: 👇
➡ The research investigates deep learning's capacity to forecast the volatility of several major assets, emphasizing the transition from simple models to more sophisticated neural networks, including the Recurrent Neural Networks and Temporal Fusion Transformer.
➡ Among the examined models, the Temporal Fusion Transformer stands out, consistently outperforming both classical approaches and simpler neural networks, indicating a significant advancement in volatility prediction accuracy.
➡ The comprehensive evaluation, encompassing performance comparisons and statistical significance testing, confirms the advanced models' effectiveness, encouraging their application in financial practices.
➡ This study's findings highlight the importance of utilizing deep learning for volatility forecasting in finance, pointing towards a shift from traditional GARCH models to more dynamic and complex neural network architectures for improved prediction accuracy.
“Return Predictability: Accounting versus Market Information”

Despite research typically favoring market-based predictors like price trends and liquidity for stock return forecasts, accounting-based predictors have been found to outperform market-based ones for predictions extending beyond one month.
This advantage, consistent across different sizes and liquidity levels and more pronounced in recent years, is attributed to the success of accounting-based strategies for longer horizons, likely due to enduring biased earnings expectations despite short-sale constraints
Simplified takeaways: 👇
➡ Accounting data outperforms market-based indicators for stock return predictions beyond a month.
➡ Machine learning analysis from 1963-2022 confirms the superior performance of accounting-based trading strategies.
➡ The study suggests biased earnings expectations contribute to accounting information's predictive edge.
➡ Accounting data's predictive power holds across different market periods, firm sizes, and liquidity levels, challenging efficient market theories.
AI-Essentials
I recently discovered this "long tutorial" on autoencoders that, in my view, is one of the most comprehensive and detailed tutorials on the topic freely available on the internet. Enjoy! 👇
Quant Finance Insights
“Regime-Aware Asset Allocation: a Statistical Jump Model Approach”
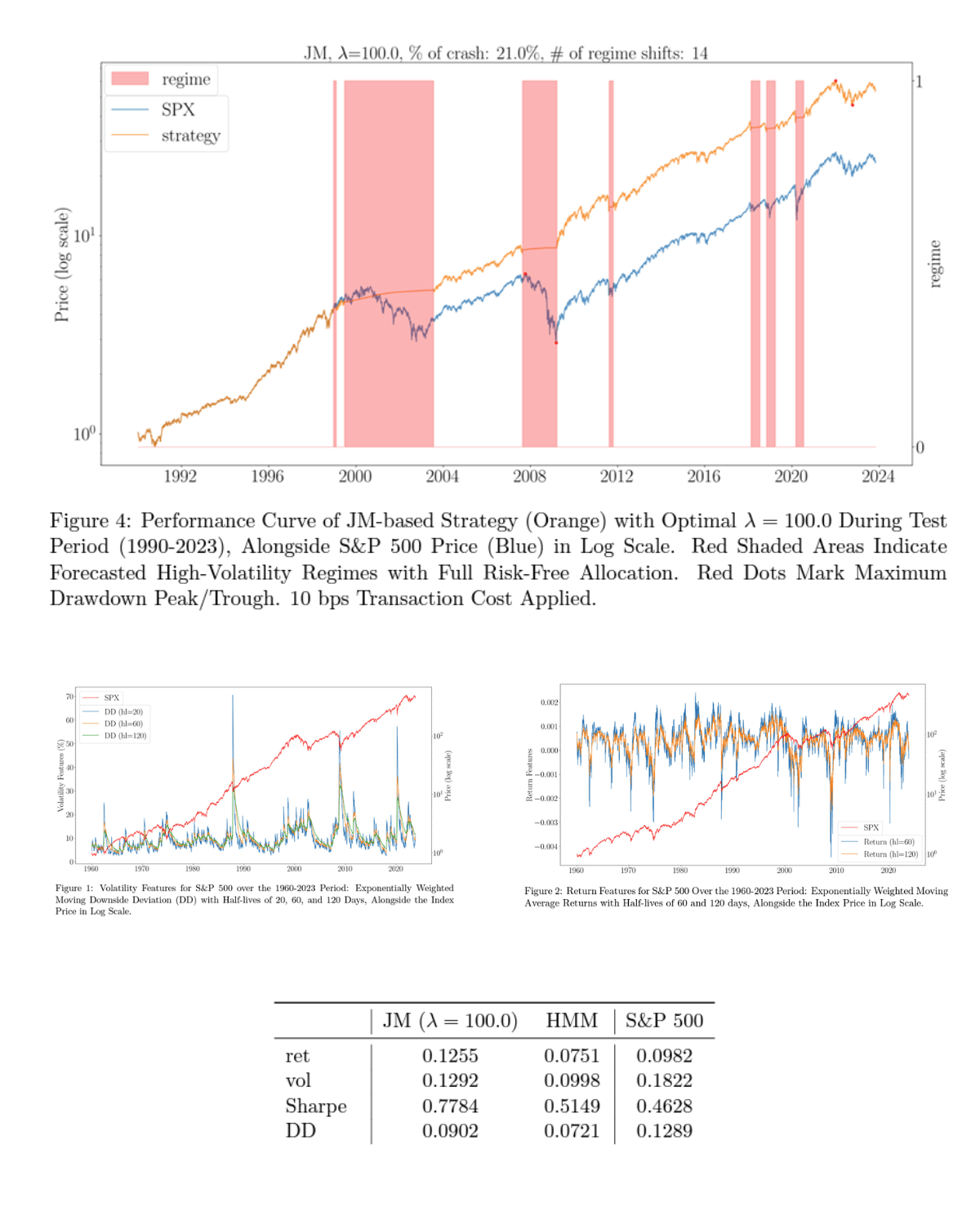
Market regime detection is one of the best applications of ML/DL in my experience. ML/DL can detect major crashes, arguably the most successful application of these methodologies for real-life investment strategies.
However, there are also many statistical-based methods that we can combine or use alone with promising results and easier traceability (with stronger links to sound financial theory), as proposed in the paper.
Here are my simplified takeaways from the paper: 👇
➡ The paper looks at how switching between market conditions affects where people choose to invest. It compares old methods with a new model called the statistical jump model. This new model is better at spotting long-lasting market trends because it uses a special penalty for jumps.
➡ The jump model uses price changes and market volatility to work. It picks the jump penalty by looking at past data, helping to make better investment strategies with a step-by-step method.
➡ A study of daily market returns from big US stock indices shows that the jump model does better than both the old buy-and-hold method and previous switching models. This means the jump model is more reliable, clear, and realistic for planning where to invest.
➡ These results are useful for investment managers. They show the benefits of using jump models to make smarter investment choices and enhance how investment strategies are made.
If you're enjoying our newsletter and want to support us, please recommend it to anyone you know who's interested in AI and Finance. Your referrals are the biggest compliment and help us grow! 🌟🤖💼