- The AI Finance Frontier
- Posts
- Can AI Read the Minds of Corporate Executives?
Can AI Read the Minds of Corporate Executives?
ML/DL insights and applications to real estate investment portfolios and probability of default prediction.

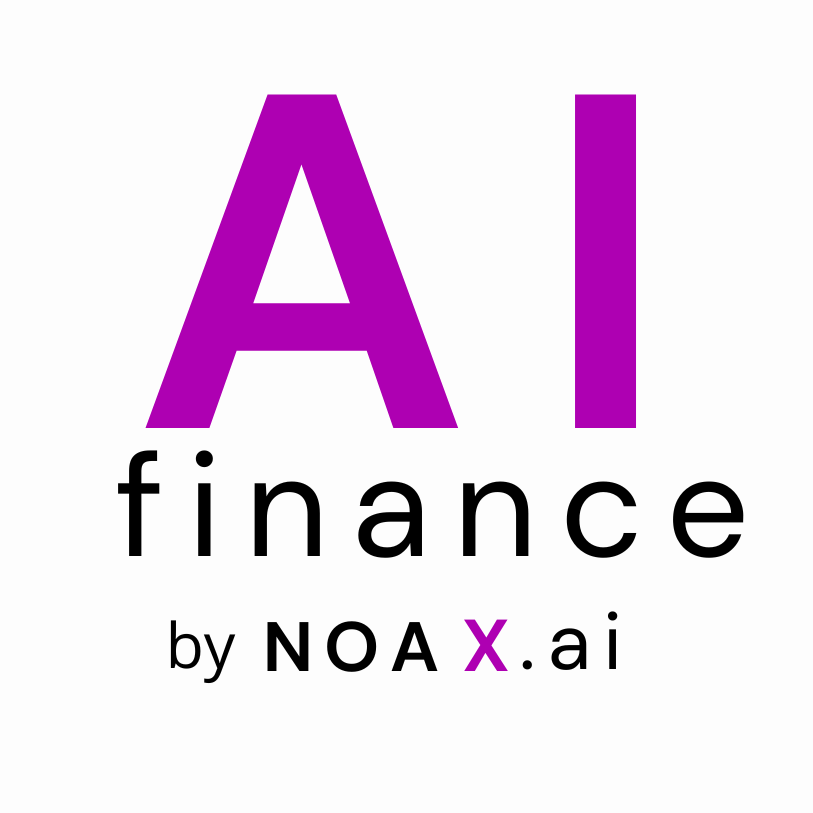
Hi! Here's Iván with this week's exciting newsletter, brimming with insights and discoveries on building robust risk models and trading strategies using Machine Learning. This edition is notable for its emphasis on the application of ML/DL to real estate investment and risk prediction.
🕹️ 2 Academic Articles: Dive into groundbreaking research featuring actionable ideas that are reshaping our understanding of how to apply ML/DL in creating successful investment and trading strategies.
💊 Video Insight : Great introduction to using neural networks for modeling the probability of default.
✔️ Market Insight: Best market reflection shared on my LinkedIn/Twitter during the last week: the financial market is always first!
🥐 Credit Default Prediction using DL: More advanced risk management application to deepen your understanding of how to design robust ML/DL risk models.
Academic Insights
“Can AI Read the Minds of Corporate Executives?”

Summary of the paper in only 5 ideas: 👇
Only Large Language Models (LLMs) trained with finance-specific objectives successfully predict both positive and negative earnings surprises and future firm returns by understanding past 10-Q and 10-K contexts.
Classic machine learning approaches, including sentiment-based and bag-of-words models, fail to forecast future earnings based on past managerial discussions.
Analysis of U.S. corporations' filings reveals that the length of the MD&A ("Management Discussion and Analysis") section is negatively associated with future earnings surprises and firm returns.
These AI models uncover significant, often complex, informational content in corporate filings, surpassing both human analysts and traditional NLP methods in performance.
The study shows the advanced ability of recent AI models to detect crucial information hidden in the intricate details of publicly disclosed corporate documents.
“Boosting Real Estate Returns with Machine Learning”

Let me summarize the 5 main takeaways of the paper: 👇
The authors test 5 machine learning algorithms (ML), including Ordinary Least Squares Linear Regression (LR), Support Vector Regression (SVR), k-Nearest Neighbors Regression (KNN), Extreme Gradient Boosting (XGBoost), and Long/Short-Term Memory Neural Networks (LSTM).
The algorithms are applied to predict future prices for 30 REITs from the US, UK, and Australia, as well as 30 stocks and 30 bonds.
They compare the ML results with other financial model benchmarks, such as Holt’s Exponential Smoothing (HES), Trigonometric Seasonality, Box–Cox Transformation, ARMA Errors, Trend, and Seasonal Components (TBATS), and Auto-Regressive Integrated Moving Average (ARIMA).
The assets were then used as part of a portfolio, which they optimized using a genetic algorithm.
Results showed that using ML algorithms for price prediction provided at least three times the return over benchmark models and reduced risk by almost two-fold. In particular, results indicated that SVR was the best-performing algorithm in terms of risk-adjusted returns across different time horizons, as confirmed by the Friedman test results (Sharpe ratio).
AI-Essentials: Step-by-Step Tutorial
🚀 In today's newsletter, we're excited to share a video that offers an excellent introduction to using neural networks for modeling the probability of default.
The post: Market Ideas
Long-term trends show the S&P 500 and gold often swing wildly in returns. They're closely linked to stock valuation, with periods where stocks beat gold, followed by decades where gold outperforms stocks 👇

ML Risk: Insight
“Improving Credit Default Prediction with Deep Learning”

The paper proposes a new feature generation methodology that improves the performance of ML/DL credit default models. Let me try to sum up the main ideas of the paper as an excuse to highlight the importance of feature generation in any ML/DL model. 👇
Traditional machine learning methods for credit default prediction, such as Logistic Regression, Support Vector Machine, and Decision Trees, use a linear combination of a few features for forecasting. These models are simple and easy to implement, and have proven effective in credit sector classification tasks.
Researchers have noted the limitations of traditional single-classifier methods in capturing complex non-linear relationships and ensuring robustness.
Feature generation, creating new features from existing ones, is crucial for enhancing data's expressive power. Various approaches have been explored: (a) The FM model uses latent vectors to capture feature interactions, bypassing manual attribute relationship analysis. (b) Deep Neural Networks (DNN), like Factorization Machine Supported Neural Network (FNN) or Product-Based Neural Network (PNN), interpret complex feature interactions. However, these models often overlook lower-order components and the model's overall weight distribution.
This study introduces an adaptive feature cross-compression method for credit default prediction. Utilizing the SENET gating mechanism, it eliminates data interference and adaptively obtains weighted embedded features. The cross-compression unit captures latent interactions between original and weighted embedded features, thus enhancing user discrete feature representation.
To verify the effectiveness of the proposed method, experiments were conducted on two real-world datasets provided by the Tianchi and Lending Club platforms. The performance was compared with 11 different predictive methods, including LR, XGBoost, and FM. The results indicate that the proposed method enhanced AUC performance by 1-2% and increased KS by 0.5-4.5%.
If you're enjoying our newsletter and want to support us, please recommend it to anyone you know who's interested in AI and Finance. Your referrals are the biggest compliment and help us grow! 🌟🤖💼