- The AI Finance Frontier
- Posts
- Can ChatGPT Beat Wall Street?
Can ChatGPT Beat Wall Street?
Unveiling the Potential of AI in Stock Selection

Hi! Here's Iván with this week's exciting newsletter, brimming with insights and discoveries on building robust investment strategies and risk models using Machine Learning.
In this edition, I am presenting the following sections:
🕹️ AI-Finance Insights: I summarize three must-read academic papers that mix cutting-edge ML/DL with Quant Finance:
Can ChatGPT Beat Wall Street? Unveiling the Potential of AI in Stock Selection
Deep Learning for Corporate Bonds
Deep Learning from Implied Volatility Surfaces.
💊 AI Essentials: The section on top AI & Quant Finance learning resources: Today, I'm excited to share a video course that is almost two hours long, guiding you step by step through the main mathematical concepts for machine learning. If you're wondering which fundamental concepts you should start with to gain a deeper understanding of machine learning, this video is for you.
🥐 Asset Pricing Insights: In this edition, I recommend a paper exploring the feasibility of successfully timing the market portfolio using a wide range of well-known inputs.
Today’s Sponsor
Rise and grind, dollar bills!
Put your money to work in a high-yield cash account with up to $2M in FDIC† insurance through program banks.
Get started today, with as little as $10.
AI-Finance Insights
“Can ChatGPT Beat Wall Street? Unveiling the Potential of AI in Stock Selection”
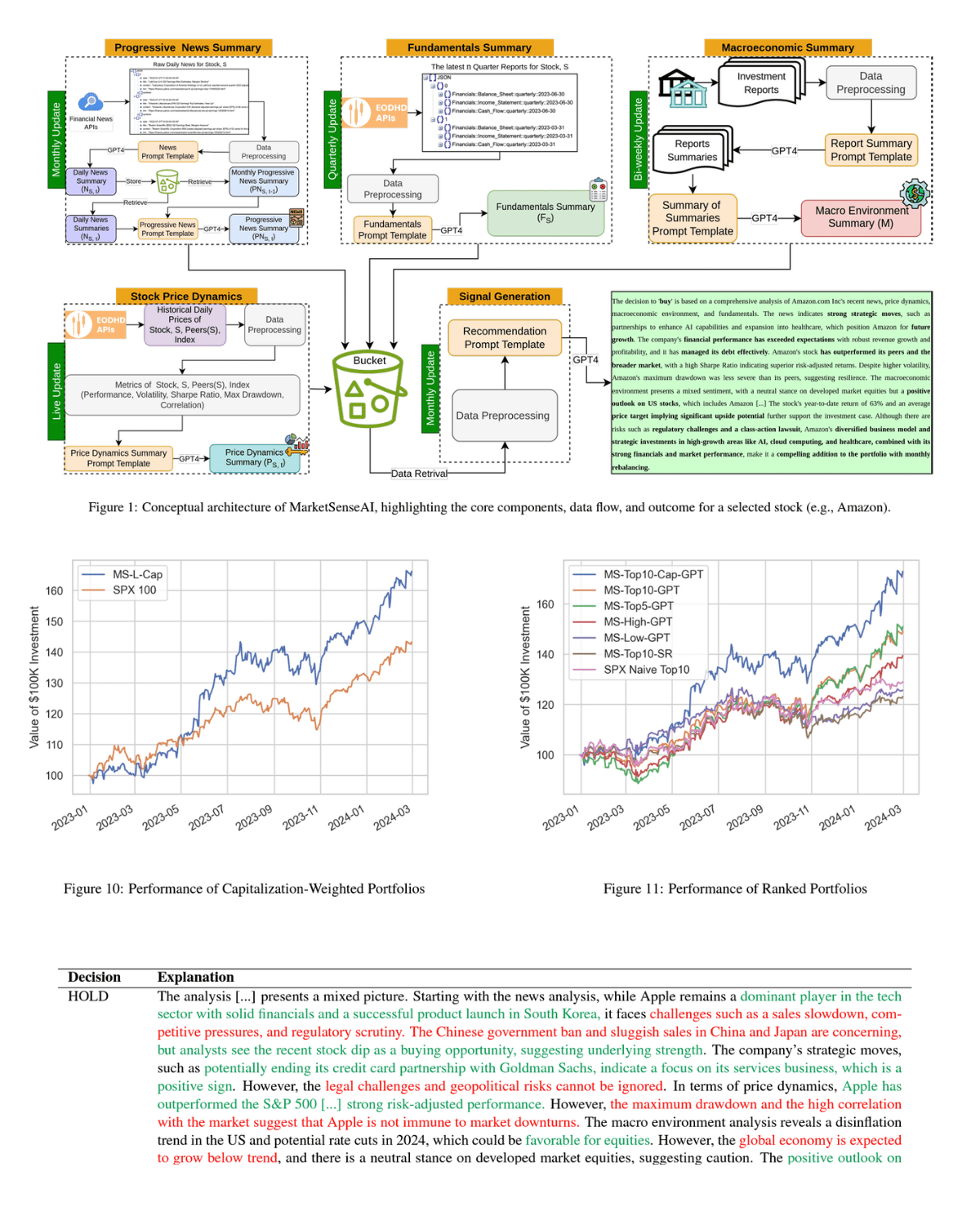
Another paper that uses ChatGPT as a tool to efficiently extract market sentiment from financial reports to build trading strategies.
Despite some important methodological limitations (most of them in the strategy section), the paper still provides a new set of ideas worth considering.
What do you think? Is it too good to be true?
...my takeaways from the paper 👇
➡ The paper introduces MarketSenseAI, a framework leveraging GPT-4 to select stocks based on Chain of Thought and In-Context Learning. By analyzing diverse data sources, it aims to replicate expert investment decision-making, using GPT-4 for prediction and signal evaluation.
➡ MarketSenseAI demonstrated excess alpha between 10% to 30% and cumulative returns of up to 72% in a 15-month test on S&P 100 stocks. The framework showcases the potential of AI in financial decision-making.
➡ Strategies using GPT-4 for ranking exhibited superior performance in total and risk-adjusted returns. MS-Top5-GPT and MS-Top10-GPT strategies achieved nearly 50% returns after costs, outperforming traditional benchmarks and showing high Sharpe and Sortino ratios.
➡ The GPT-4 ranking mechanisms demonstrated a clear advantage over traditional methods, as evidenced by the MS-Top10-Cap-GPT strategy achieving a 72% return after costs.
➡ Overall, the findings highlight the potential of integrating GPT-4 and large language models into financial strategies, providing data-driven insights for asset management.
“Deep Learning for Corporate Bonds”
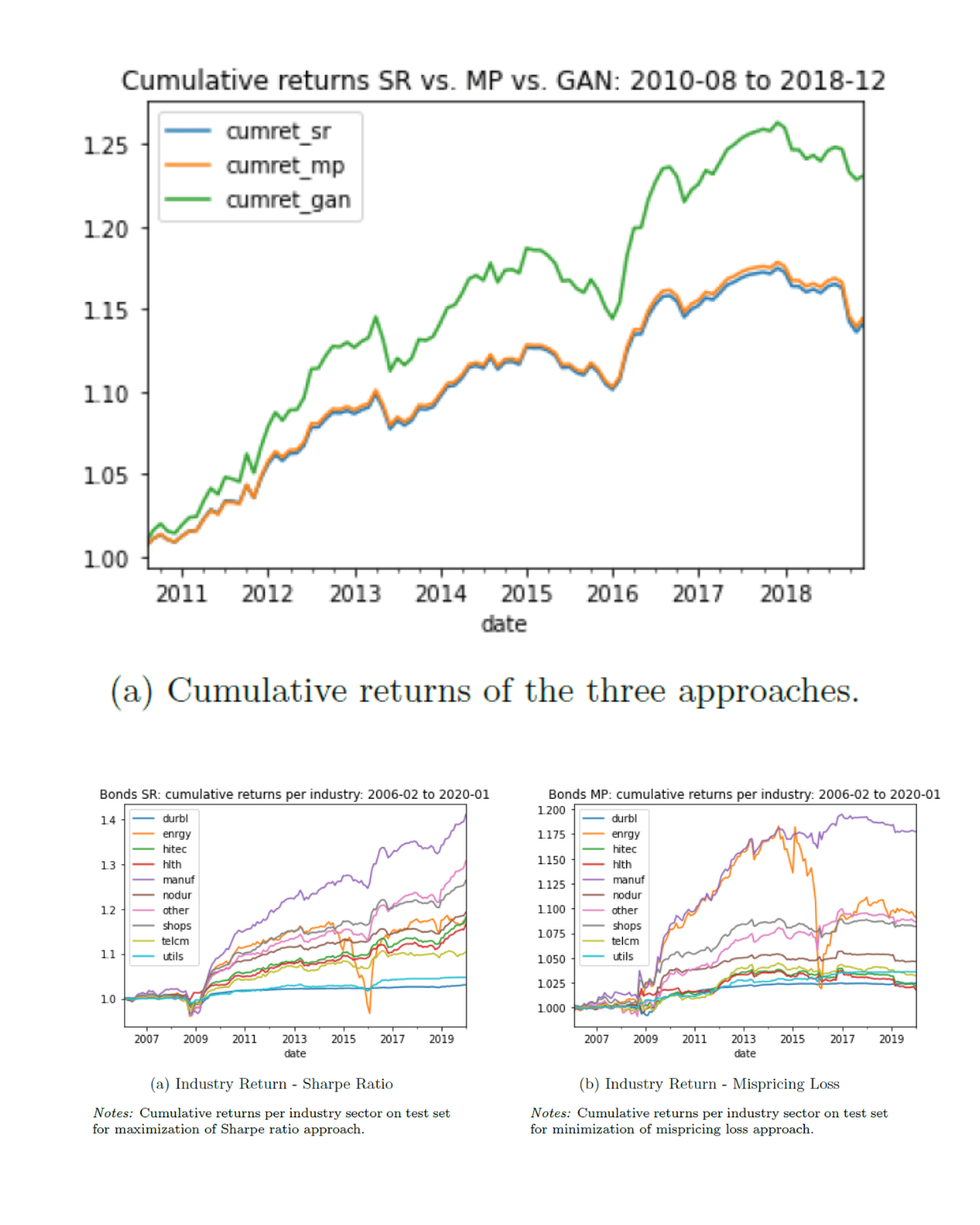
The use of deep learning in fixed income strategies is underrepresented in the literature but still deserves our attention due to the promising results of a few papers.
My takeaways from the paper:
➡ The paper explores asset pricing models for U.S. corporate bonds using advanced techniques that blend individual bond data with issuer characteristics and an extensive set of macroeconomic time series. This approach utilizes neural networks to flexibly model the stochastic discount factor (SDF), significantly diverging from traditional models that typically assume linearity in pricing factors.
➡ A key finding is the performance difference between maximizing Sharpe ratios and minimizing mispricing losses, especially highlighted in individual bonds compared to bond portfolios. This suggests that approaches maximizing the Sharpe ratio are more effective for individual assets, while the impact is less pronounced in portfolios.
➡ The research demonstrates robust out-of-sample performance with annual Sharpe ratios ranging from 0.59 to 1.00 and significant excess returns over conventional risk factors. The models incorporating a broader set of macroeconomic time series exhibit better overall results, indicating a crucial role for comprehensive macroeconomic data in enhancing model accuracy and reducing maximum drawdown.
➡ For bond portfolios, the out-of-sample Sharpe ratios lie between 0.76 and 0.87, depending on the data and model objectives. This indicates that the models, while complex, maintain practical viability under various market conditions, including constraints like maximum allowable leverage, which remains modest across models.
➡ Overall, the paper challenges traditional asset pricing theories by demonstrating that sophisticated, data-rich models can achieve higher returns with significant risk adjustment. These findings underscore the potential of neural network architectures in refining asset pricing strategies and improving predictive power in financial markets.
“Deep Learning from Implied Volatility Surfaces”

Is there any information on volatility surface images for the prediction of the cross-section of stock returns? The paper finds that using image recognition, we can enhance the prediction of stock returns.
My takeaways from the paper (links at the end of the post) 👇
➡ The study introduces a novel method that uses image recognition techniques from machine learning to analyze option implied volatility (IV) surfaces, enhancing the predictive power for stock returns.
➡ It underscores the value of ensemble machine learning models in financial predictions, showing that increasing the number of models in the ensemble directly correlates with higher out-of-sample performance.
➡Ensembles significantly improve the Sharpe ratio, from 0.9 to 2.7 in the most complex models, and from 0.80 to 1.6 in simpler models, clearly outperforming traditional methods.
➡ The research highlights that the predictive information extracted from IV surfaces is mostly uncorrelated with other existing option-implied characteristics, providing a unique and valuable perspective that delivers higher investor returns with significant alpha relative to a comprehensive set of standard and option-implied factors.
➡ Additionally, the findings are robust to practical trading conditions including short-sale constraints and transaction costs, suggesting that this methodology is not only theoretically effective but also practically applicable in real-world trading scenarios.
➡ Overall, the paper presents compelling evidence that sophisticated ML techniques, particularly deep learning applied in novel ways to financial data, can substantially improve investment strategies without increasing risk, offering a potent tool for asset managers in complex market environments.
AI-Essentials
Wondering which fundamental mathematical concepts you should start with to gain a deeper understanding of machine learning? The following video course, almost two hours long, guides you through the main math concepts for ML step by step. 👇
Sponsor 👇
AI won’t take your job, but a person using AI might. That’s why 500,000+ professionals read The Rundown – the free newsletter that keeps you updated on the latest AI news and teaches you how to apply it in just 5 minutes a day.
Asset Pricing Insights
“Managing the Market Portfolio”

Is it possible to time the market? Do not miss the following paper! 👇
Main ideas from the paper in 2 min (link at the end of the post):
➡ The paper examines the use of a comprehensive set of financial, macroeconomic, and technical variables for time-series management of market portfolios, emphasizing the enhanced predictability and return on investments it offers for multi-factor investing.
➡ By aggregating out-of-sample market excess return forecasts from these variables, the study shows how dynamic portfolio allocation can significantly exceed traditional strategies, producing alphas over 5% per annum, which underscores the effectiveness of this method in optimizing portfolio returns over long-term investment horizons.
➡ The research finds a generally weak but complex positive relationship between time-series predictive measures and multifactor alphas, with a particular emphasis on the superior importance of return predictability over volatility forecasting. This insight aids in fine-tuning investment strategies to maximize returns.
➡ Highlighting the practical application, an initial investment of $1 in 1972 in the managed market portfolio would grow to $85.84 by 2019 using this strategy, outperforming the $15.03 from a standard buy-and-hold strategy, both with similar market exposures but differing substantially in returns due to strategic management.
➡ Overall, the paper's findings advocate for the application of sophisticated data aggregation and dynamic allocation techniques in managing portfolios, which not only enhance returns but also maintain robust performance across varying market conditions, thereby offering a powerful tool for asset managers in complex investment landscapes.
If you're enjoying our newsletter and want to support us, please recommend it to anyone you know who's interested in AI and Finance. Your referrals are the biggest compliment and help us grow! 🌟🤖💼