- The AI Finance Frontier
- Posts
- Deep Learning for Market Trend Prediction
Deep Learning for Market Trend Prediction
Using Deep Learning to go beyond traditional trend-following methods.

Hi! Here's Iván with this week's exciting newsletter, brimming with insights and discoveries on building robust investment strategies and risk models using Machine Learning.
In this edition, I am presenting the following sections:
🕹️ AI-Finance Insights: I summarize two must-read academic papers that mix cutting-edge ML/DL with Asset Pricing & Quant Finance:
Deep Learning for Market Trend Prediction.
Predicting Anomalies.
💊 AI Essentials: The section on top AI & Quant Finance learning resources: Today, I’m sharing an insightful video on "Machine Learning Algorithms for Financial Markets." This talk offers a comprehensive introduction to the use of machine learning in financial markets, perfect for those looking to enhance their knowledge. It covers essential algorithms and tools, explores intraday trading strategies, and finishes with a real-world case study. If you're new to machine learning in finance or want to deepen your understanding, this is a must-watch!
🥐 Asset Pricing Insights: In this edition, I introduce “Factor Timing with Portfolio Characteristics", a paper that explores how improving factor investing strategies can lead to better returns. The authors present a novel approach to timing factor portfolios using portfolio characteristics, offering fresh insights into dynamic factor investing and portfolio management.
Today’s Sponsor
🦾 Master AI & ChatGPT for FREE in just 3 hours 🤯
1 Million+ people have attended, and are RAVING about this AI Workshop.
Don’t believe us? Attend it for free and see it for yourself.
Highly Recommended: 🚀
Join this 3-hour Power-Packed Masterclass worth $399 for absolutely free and learn 20+ AI tools to become 10x better & faster at what you do
🗓️ Tomorrow | ⏱️ 10 AM EST
In this Masterclass, you’ll learn how to:
🚀 Do quick excel analysis & make AI-powered PPTs
🚀 Build your own personal AI assistant to save 10+ hours
🚀 Become an expert at prompting & learn 20+ AI tools
🚀 Research faster & make your life a lot simpler & more…
“Deep Learning for Market Trend Prediction”

👉 This paper argues for leveraging ML to predict future market trends, improving asset management by increasing returns and reducing risks compared to standard trend-following strategies. The authors advocate for AI-driven approaches that consider non-linear relationships in financial data, unlike traditional linear models.
👉 Deep learning models, like Artificial Neural Networks, have shown a capacity to differentiate non-linear trends, capturing market dynamics that simpler linear models fail to recognize. By integrating contextual data—such as economic indicators and diverse financial market data—deep learning models provide a nuanced understanding of potential market movements.
👉 The research finds that using a nonlinear model, particularly during market shocks like the COVID-19 pandemic, allows for a faster and more accurate reaction compared to linear methods. These models adapt to the rapid shifts in market trends, which could give asset managers an edge over competitors relying solely on historical trend-following.
👉 The authors emphasize that these ML/DL tools are designed to support, not replace, human asset managers. Risk indicators developed using deep learning guide portfolio adjustments, enhancing decision-making under complex market conditions.
“Predicting Anomalies”

It is possible to leverage anomaly signals before they’re public! Keep reading!🔻
👉 This paper argues that stock returns follow predictable patterns even before anomaly trading signals are released! The authors reveal that anomalies themselves are predictable, offering traders an opportunity to act before financial statements hit the news. This preemptive trading could unlock additional returns!
👉 They show that by predicting these signals, traders can earn an extra 3.65% annualized return in the quarter before the signals are publicly available. This strategy works especially well for anomalies that are harder to forecast, as the market hasn’t yet priced in this information.
👉 The authors test several models—from machine learning to simple autoregressive models—and find that a martingale model (predicting next signals based on current trends) often performs best, with an impressive F1 score of 70.2%!
👉 Takeaway: Predicting anomalies isn’t just about looking at past performance....it’s about identifying persistent trends.
Sponsor 👇
The Biggest Disruption to $martphones Since iPhone
Over the last 3 years, Mode has seen 32,481% Growth, making them one of America’s fastest growing companies. Mode is on a mission to disrupt the entire industry with their “EarnPhone”, a budget smartphone that’s helped consumers earn and save $325M for activities like listening to music, playing games, and… even charging their devices?!
Mode has over $60M in revenue - this is your chance to invest in a $1T+ market opportunity!
This is a paid advertisement for Mode Mobile Reg A offering. Please read the offering statement at https://invest.modemobile.com/.
AI-Essentials
In this edition, we're featuring "Machine Learning Algorithms for Financial Markets." This talk begins with an overview of essential algorithms used in financial markets and introduces key machine learning tools. It then explores how these technologies can be applied to intraday trading strategies, concluding with a real-world case study.
Asset Pricing Insights
“Factor Timing with Portfolio Characteristics”
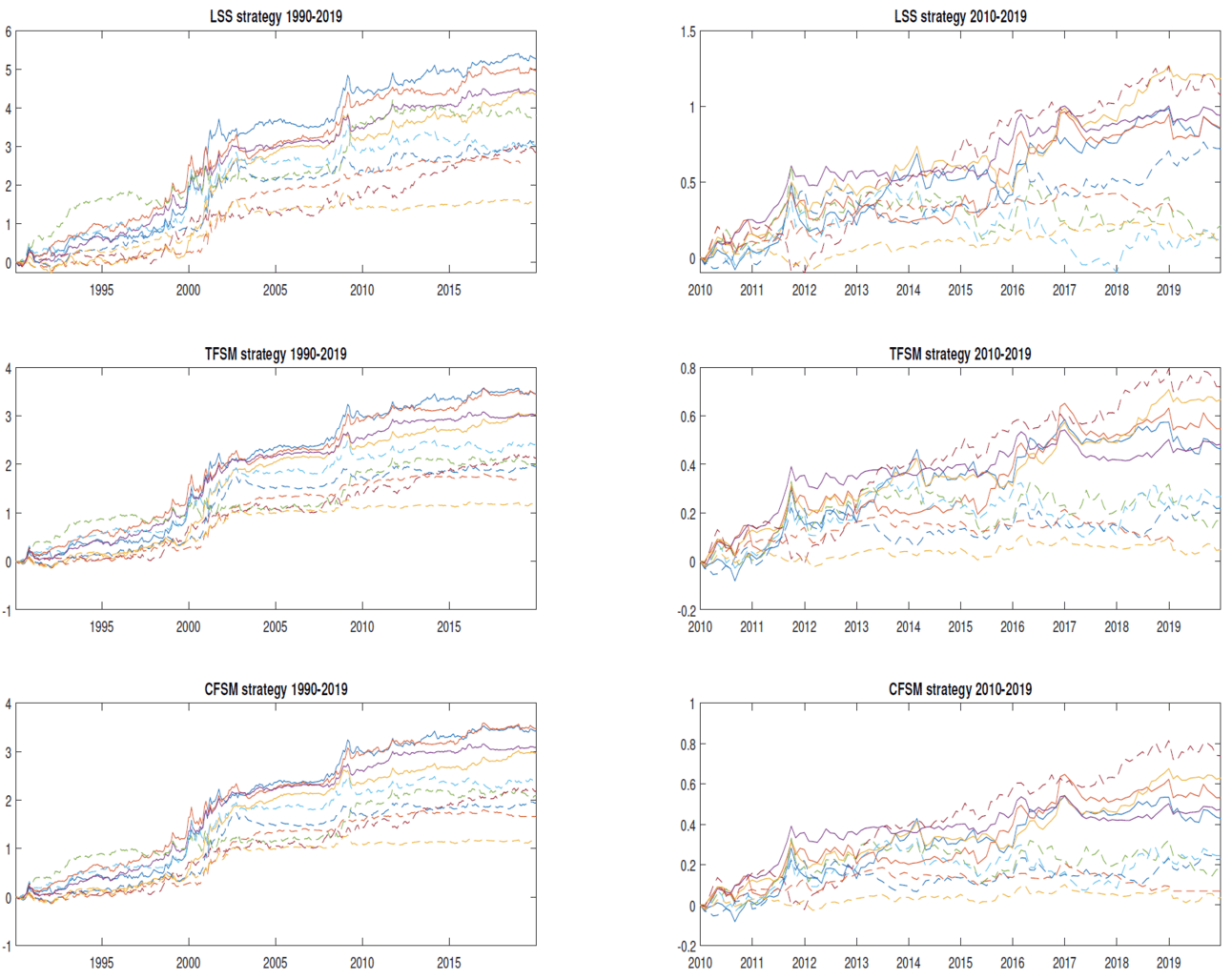
📢New Asset Pricing Ideas! "Factor Timing with Portfolio Characteristics" how to improve a factor investing strategy? This paper suggests a unique way to time factor portfolios using portfolio characteristics. Let’s break it down! 🔻
👉 The authors introduce a new approach to predict factor portfolio returns using aggregated portfolio characteristics. Instead of static strategies, their method uses characteristic-based models to actively time factor returns and maximize gains.
👉 Using techniques like dimension reduction and LASSO, the paper finds that predicting factor portfolios using characteristics delivers superior performance! It outpaces existing models in both accuracy and return prediction, with annualized returns of up to 1.47% and Sharpe ratios of 0.73.
👉 Methodology:
➡ They apply Principal Component Analysis (PCA) and Partial Least Squares (PLS) to compress and focus on key predictive information.
➡By combining portfolio characteristics, the model captures important trends for factor timing, giving it an edge over static factor investing.
If you're enjoying our newsletter and want to support us, please recommend it to anyone you know who's interested in AI and Finance. Your referrals are the biggest compliment and help us grow! 🌟🤖💼