- The AI Finance Frontier
- Posts
- Generative Machine Learning for Multivariate Equity Returns
Generative Machine Learning for Multivariate Equity Returns
Conditional importance-weighted autoencoders modeling equity returns.
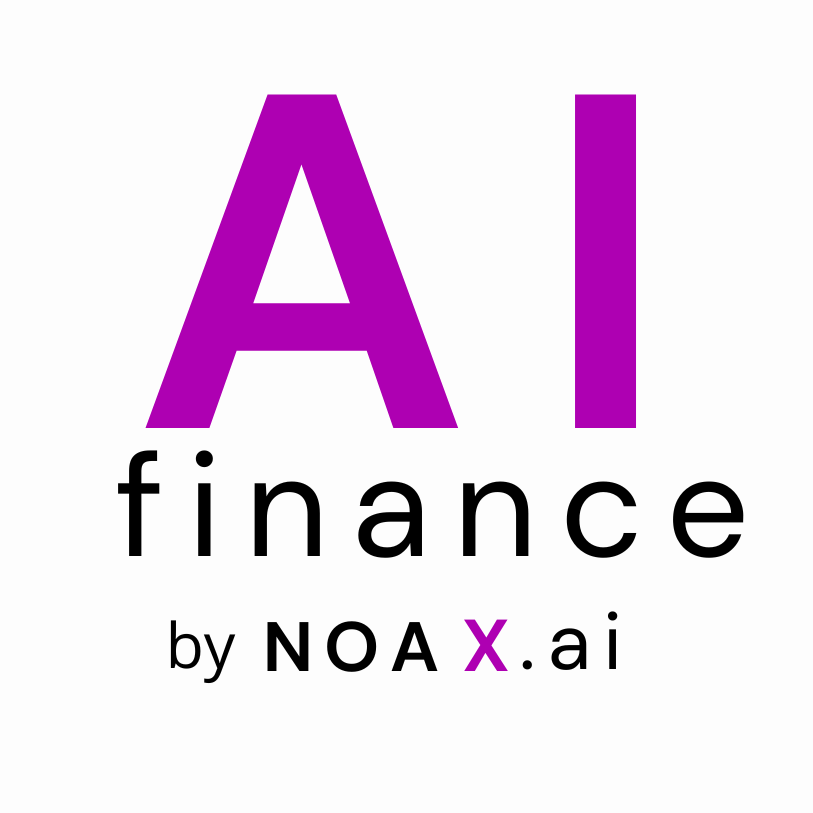
Hi! Here's Iván with this week's exciting newsletter, brimming with insights and discoveries on building robust investment strategies and risk models using Machine Learning.
In this edition, I am presenting the following sections:
🕹️ AI-Finance Insights: I summarize three must-read academic papers that mix cutting-edge ML/DL with Quant Finance:
Generative Machine Learning for Multivariate Equity Returns
A Deep Reinforcement Learning Based Automated Stock Trading System Using Cascaded LSTM Networks
Empirical Asset Pricing with Probability Forecasts
💊 AI Essentials: The section on top AI & Quant Finance learning resources: Today, I am sharing a talk by Marcos Lopez de Prado about causal factor investing that is a must-watch to understand this new stream of research.
🥐 Quant Finance Insights: In this edition, I share a relevant paper on how to apply risk-adapted momentum strategies to crypto investments.
Today’s Sponsor
Shift Left: How to Turn Security into Review
In the competitive landscape of software business, optimizing processes and leveraging efficiencies can make a significant difference in building a strong pipeline and closing revenue faster.
Download the ebook from Vanta to learn how to:
Apply the DevOps principles of “shifting left” to position security as a differentiator — instead of a hurdle
Center security in your sales conversations at every stage to proactively remove roadblocks to revenue
Invest in your security story by making it easy for buyers to access security-related information
Vanta helps SaaS businesses of all sizes manage risk and prove security in real time.
AI-Finance Insights
“Generative Machine Learning for Multivariate Equity Returns”

Leveraging generative models for synthetic data is becoming essential in finance. These models learn the data's distribution, akin to traditional statistical modeling.
This paper examines the effectiveness of modern machine learning techniques, particularly conditional importance-weighted autoencoders and conditional normalizing flows, in modeling equity returns.
My sum up in under 3 min: 👇
➡ The paper addresses the critical importance of risk forecasting in finance for estimating portfolio risk, volatility, and correlation, and its role in portfolio optimization, using a deep learning approach to develop a statistical model that learns the multivariate distribution of stock returns.
➡ It contrasts traditional methods that focus on individual statistical measures (like quantiles for Value at Risk, or VaR) with comprehensive statistical models such as GARCH, which aim to learn the entire distribution of returns, thus enabling queries of any statistical quantity from the learned distribution.
➡ The research investigates the utility of deep probabilistic models, including variational autoencoders and normalizing flows, for modeling the distributions of returns, proposing a novel approach to predict stock returns as an arbitrary probability distribution.
➡ This work attempts to bridge a gap in existing literature by modeling the joint distribution of an arbitrary number of stocks, a task not previously accomplished, highlighting the importance of capturing correlations between stock returns for accurate risk forecasting.
➡ The methodology outlined includes the use of two innovative models: a conditional importance-weighted autoencoder (CIWAE) for broader market factors, and a conditional normalizing flow for individual stock returns, showcasing a forward-thinking approach to financial modeling.
➡ The inclusion of borrowing costs in portfolio optimization, set to 0.1 basis points (bps) daily based on prior work's annual rate of 17 bps, demonstrates nuanced financial modeling. Results show that including borrowing costs for an optimal portfolio slightly reduces the Sharpe ratio from 2.52 to 2.42.
➡ However, incorporating borrowing costs into the optimization process slightly improves the Sharpe ratio, indicating the model's sensitivity and adaptability to financial assumptions.
➡ Finally, the paper emphasizes the practical applications of its methodology, including enhanced risk estimation for individual securities and portfolios, and the creation of portfolios that outperform the market, demonstrating the potential of neural networks as a competitive alternative to classical estimators in finance.
“A Deep Reinforcement Learning Based Automated Stock Trading System Using Cascaded LSTM Networks”

The paper employs deep reinforcement learning to develop automated stock trading strategies that maximize expected returns across multiple stocks. It treats stock trading as a Markov decision process, utilizing states, actions, rewards, and strategies to learn how to optimize the value function without market direction labels.
The training uses the Proximal Policy Optimization (PPO) algorithm and Long Short-Term Memory (LSTM) to analyze time-series data, including stock prices, balance, shares, and technical indicators.
My sum up in under 3 min: 👇
➡ The abstract highlights the growing use of deep reinforcement learning (DRL) algorithms in constructing stock trading strategies, noting the adaptation challenges these methods face when applied to financial data characterized by low signal-to-noise ratios and unevenness, which often lead to performance issues.
➡ To address these challenges, the paper proposes a DRL-based stock trading system utilizing a cascaded LSTM (CLSTM-PPO Model). This model initially employs LSTM to extract time-series features from daily stock data, after which these features are used to train the agent. A separate LSTM is also used within the strategy functions for reinforcement learning training.
➡ The effectiveness of the proposed model is demonstrated through experiments involving 30 stocks each from four major stock indexes: the Dow Jones Industrial Index (DJI) in the US, SSE50 on the Shanghai Stock Exchange in China, SENSEX on the Bombay Stock Exchange in India, and FTSE 100 on the London Stock Exchange in the UK.
➡ Results show that the model surpasses previous baseline models in terms of cumulative returns (5% to 52% improvement), maximum earning rate (8% to 52% increase), and average profitability per trade (6% to 14% enhancement).
➡ The abstract concludes that the proposed method is a promising approach to building an automated stock trading system, suggesting potential for significant advancements in financial trading strategies through the application of sophisticated DRL models.
“Empirical Asset Pricing with Probability Forecasts”

Instead of predicting returns, as many other papers do, this paper introduces an intriguing approach by predicting the probabilities that stocks will outperform a benchmark.
It uses a wide range of firm characteristics as predictors, akin to methods seen in ML studies.
Here are my main takeaways from the paper: 👇
➡ The study explores probability forecasts in cross-sectional asset pricing, examining a large number of firm characteristics and comparing simple and sophisticated forecasting models.
➡ Surprisingly, the findings reveal that a simple probability forecast model can perform as well as a more sophisticated one. Both types of models generate long-short portfolios with Sharpe ratios comparable to those from traditional return forecasts.
➡ Importantly, combining probability forecasts with return forecasts results in superior portfolio performance compared to using each forecast type individually. This suggests that probability forecasts offer valuable additional insights beyond return forecasts.
➡ This indicates the potential untapped value of probability forecasts in enhancing our understanding of the cross-section of stock returns, pointing to new directions for improving asset pricing models and investment strategies.
AI-Essentials
Although it is not a pure AI resource, the causal factor investing literature is gaining traction and is closely related to the application of ML/DL in investments. The following talk by Marcos Lopez de Prado is a must-watch to understand this new stream of research.
Quant Finance Insights
“Cryptocurrencies: Stylized Facts, & Risk Based Momentum Investing”

Nice risk-adapted, momentum-based strategies for crypto assets. Simple to implement and promising results as a starting point.
My sum up in under 3 min: 👇
➡ The research explores the distributional characteristics of cryptocurrencies and assesses the feasibility of risk-based and trend-following investment approaches in this asset class, noting cryptocurrencies' recent emergence compared to traditional assets.
➡ The study selects a list of cryptocurrencies for analysis from October 2017 to the present, based on yearly market cap rankings, acknowledging the implicit look-ahead and survivorship biases but focusing on understanding asset distribution and momentum feasibility.
➡ The analysis is divided into two sections: demonstrating cryptocurrency return characteristics to predict risk and persistence in returns, and analyzing the performance of three sets of strategies (risk-based, risk-based with trend following, and risk-based with trend for long-short portfolios) against a simple equal-weighted benchmark.
➡ Risk-based methods show significant improvement over the simple long-only benchmark, with higher geometric Sharpe ratios. However, there's little difference in risk-adjusted performance across four allocation frameworks.
➡ The scenario changes when trend overlays are added, significantly enhancing performance. This suggests a synergy when combining trend following with risk-based allocation.
➡ The inverse variance portfolio stands out, especially with the addition of trend overlays, outperforming the simple benchmark and the equal-weighted trend approach with a geometric Sharpe Ratio of 0.87 versus 0.68.
➡ The study also examines long-short portfolios, highlighting the inverse variance portfolio's superior performance with a Sharpe ratio of 1.50. This is notable given the impracticality of shorting cryptocurrencies at scale during the backtest periods, which could lead one to expect outsized risk-adjusted performance.
If you're enjoying our newsletter and want to support us, please recommend it to anyone you know who's interested in AI and Finance. Your referrals are the biggest compliment and help us grow! 🌟🤖💼