- The AI Finance Frontier
- Posts
- Identifying Market Regimes with Machine Learning
Identifying Market Regimes with Machine Learning
Predicting market regimes (crashes) with ML/DL
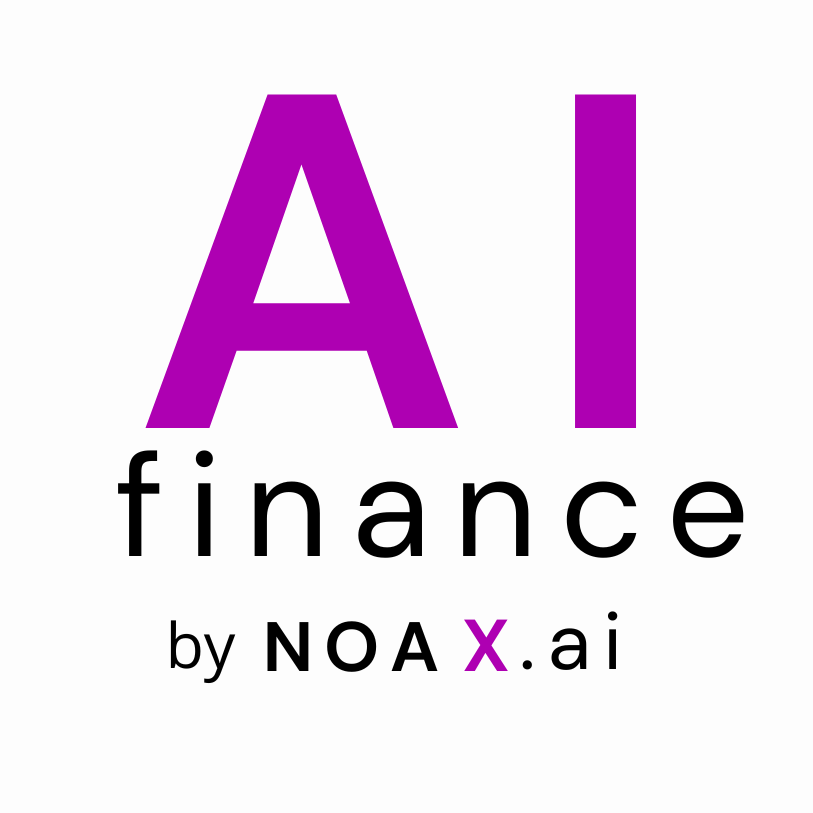
Hi! Here's Iván with this week's exciting newsletter, brimming with insights and discoveries on building robust investment strategies and risk models using Machine Learning.
In this edition, I am presenting the following sections:
🕹️ AI-Finance Insights: I summarize three must-read academic papers that mix cutting-edge ML/DL with Quant Finance:
Identifying Market Regimes with Machine Learning
State-dependent Asset Allocation Using Neural Networks
Multi-source aggregated classification for stock price movement prediction
💊 AI Essentials: The section on top AI & Quant Finance learning resources: Today, I'm introducing one of the best free courses on Deep Learning (by Yann LeCun & Alfredo Canziani).
🥐 Quant Finance Insights: In this edition, I share a novel method that enhances factor investing by applying machine learning prior to the sorting process.
Today’s Sponsor
Get the best stock ideas
Our AI tool scours the internet every day for the best stock ideas that we share with you each morning in our free, daily email.
We find stock ideas from:
Billion-dollar hedge funds
Professional analysts
Millionaire investors
and more…
We’ve already found stock ideas like:
Carvana ($CVNA) - +822% in 4 months
Myomo ($MYO) - +507% in 3 month
ImmunityBio ($IBRX) - +313% in 1 month
and a ton more…
Subscribe to our free, daily email to start getting the best stock ideas sent to your inbox each morning.
AI-Finance Insights
“Identifying Market Regimes with Machine Learning”
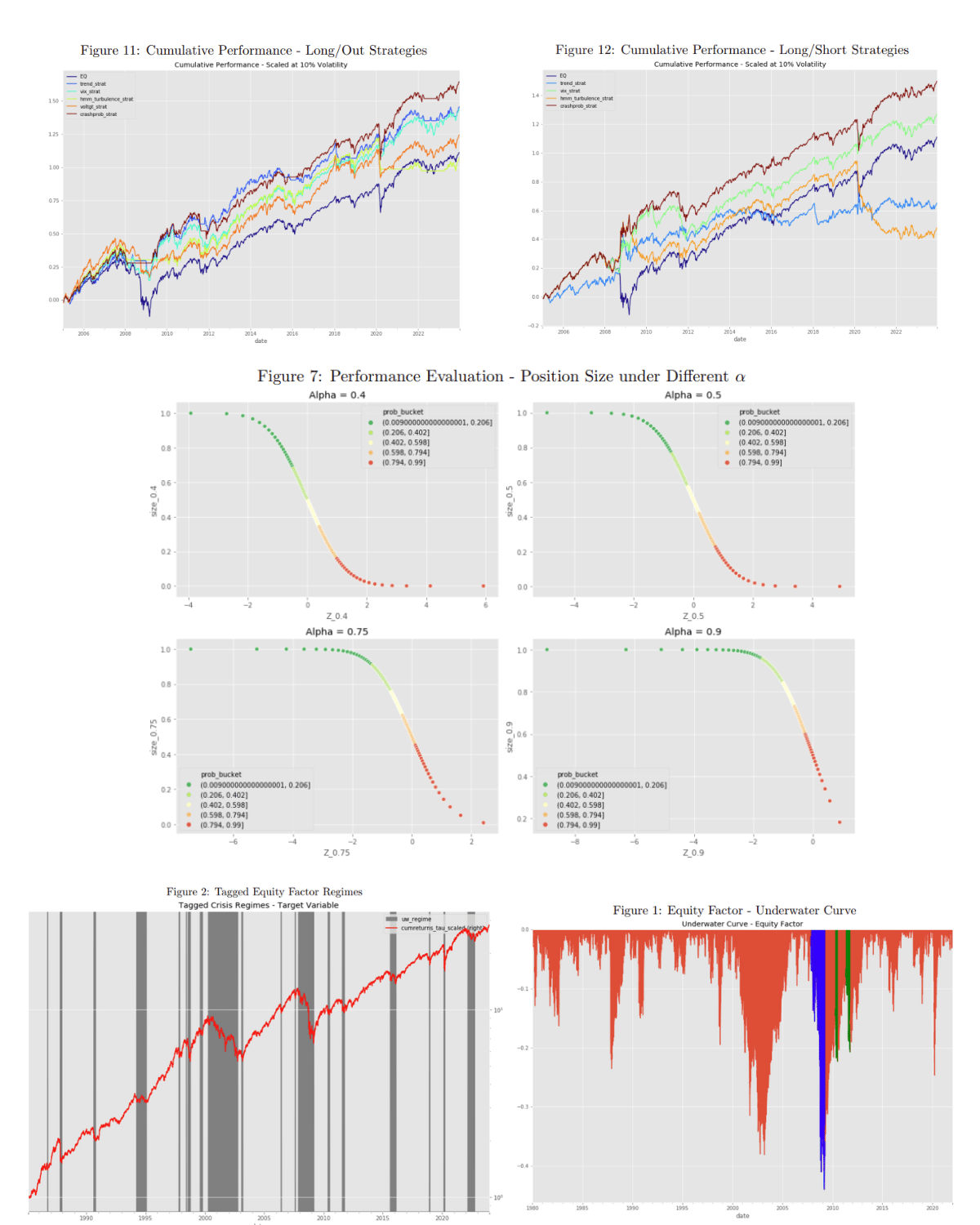
An interesting approach to predicting market regimes (crashes) that can be easily adapted to various trading strategies and asset classes as an additional step in your predictive framework.
Here are my 5 key takeaways from the study: 👇
➡ The research presents a supervised learning approach to detect regimes in financial risk factors, focusing primarily on the Equity Factor due to its significant impact on portfolio construction across both institutional and alternative investors.
➡ It highlights the importance of recognizing structural breaks or regime shifts in risk factors, which can critically influence both strategic and tactical asset allocation decisions.
➡ By predicting the crash probability of the Equity Factor—defined as the likelihood of a drawdown exceeding a specific threshold—the study identifies two distinct states of equity risk, offering valuable insights for managing exposure.
➡ The methodology utilizes a comprehensive set of market features for model estimation and demonstrates the superior performance of an ensemble model in comparison to traditional benchmarks, such as trend-following rules and hidden Markov models.
➡ The paper also explores practical applications in portfolio construction, showing how forecasts can be used to adjust risk allocation and improve risk predictions in risk-based portfolios, pointing towards innovative strategies for risk management and investment.
“State-dependent Asset Allocation Using Neural Networks”

The paper proposes an interesting approach that utilizes deep learning in conjunction with state variables to directly determine tactical portfolio weights within the optimization problem.
Here's my summary in only 3 main ideas: 👇
➡ The paper introduces a novel machine learning-based approach to conditional asset allocation that adapts portfolio allocations in response to changing market conditions without relying on the usual predictions of means and covariances.
➡ This method directly links market states to portfolio weights, bypassing the traditional step of modeling return distributions first. It's designed to capture nonlinearity between state variables and portfolio weights and doesn't assume a specific return distribution.
➡ Empirical tests on stock and bond index portfolios show this approach outperforms traditional asset allocation methods and works well across different objectives and time periods.
“Multi-source aggregated classification for stock price movement prediction”

Combining different sources of data for our ML/DL predictive models is a complex task.
This paper provides a range of interesting ideas that could help us to come up with new ways of aggregating data from different sources in a DL (Deep Learning) framework.
👇 Here are 5 key takeaways from the study:
➡ The study introduces a novel Multi-source Aggregated Classification (MAC) method, leveraging deep learning to predict stock price movements. It emphasizes the integration of numerical features and market-driven news sentiments for a comprehensive analysis.
➡ MAC method outshines traditional sentiment analysis by incorporating news sentiments of related stocks, addressing the limitation of focusing solely on target companies. This approach acknowledges the interconnected nature of stock prices among related companies.
➡ A unique feature of MAC is the use of a pre-trained embedding feature generator, which aligns news sentiments with real stock price movements, offering a more accurate representation of market sentiments in vector space.
➡ The inclusion of a graph convolutional network allows MAC to capture the nuanced effects of related companies' news on the target stock, enhancing the prediction accuracy.
➡ Through extensive experiments, MAC demonstrates superior performance over existing models in predicting next-day stock price movements, achieving better Sharpe Ratio and backtesting trading incomes. This breakthrough suggests a promising direction for future research and applications in financial market predictions.
AI-Essentials
Arguably the best free course on Deep Learning, based on YouTube videos along with code examples. It caters to everyone from beginners to advanced students... and most importantly, it's designed and taught by Yann LeCun & Alfredo Canziani.
Quant Finance Insights
“Uncertainty-Aware Lookahead Factor Models for Quantitative Investing”

Instead of sorting by factors, let's forecast future factors using deep learning and sort based on those forecasts.
Here's a quick sum up of the paper in under 2 minutes: 👇
➡ This research breaks new ground by showing that if we could pick stocks based on future financial data (think earnings, debt, etc.), those portfolios would massively outshine those selected using today's standard models.
➡ By training advanced deep learning networks, the team successfully predicts these future fundamentals based on the past 5 years of data, creating what's termed as "lookahead factor models."
➡ These models not only forecast stock performance but also incorporate risk aversion through innovative uncertainty estimates, significantly boosting portfolio returns and risk-adjusted performance (Sharpe ratio).
➡ With an industry-grade simulator, the team proves that these enhanced models can deliver an impressive annualized return of 17.7% and a Sharpe ratio of 0.84, outperforming traditional models by a significant margin.
➡ The insights challenge the conventional wisdom in quantitative finance, pointing towards a new era where predictive analytics and risk management converge to optimize stock portfolio performance.
If you're enjoying our newsletter and want to support us, please recommend it to anyone you know who's interested in AI and Finance. Your referrals are the biggest compliment and help us grow! 🌟🤖💼