- The AI Finance Frontier
- Posts
- Machine Learning and the Yield Curve: Tree-Based Macroeconomic Regime Switching
Machine Learning and the Yield Curve: Tree-Based Macroeconomic Regime Switching
How ML helps with yield curve prediction by uncovering hidden macroeconomic regimes.

Hi! Here's Iván with this week's exciting newsletter, brimming with insights and discoveries on building robust investment strategies and risk models using Machine Learning.
In this edition, I am presenting the following sections:
🕹️ AI-Finance Insights: I summarize two must-read academic papers that mix cutting-edge ML/DL with Asset Pricing & Quant Finance:
Machine Learning and the Yield Curve: Tree-Based Macroeconomic Regime Switching.
Optimal Factor Timing in a High-Dimensional Setting.
💊 AI Essentials: The section on top AI & Quant Finance learning resources: Today, I’m sharing a 40-minute video that’s perfect for total beginners: an introduction to Cursor AI. This video walks through the basics of this powerful tool, showing how even non-developers can use it to improve their workflow. If you’re new to AI and coding, this is a great starting point to explore the possibilities of Cursor AI.
🥐 Asset Pricing Insights: In this edition, I introduce “The Subjective Belief Factor”. A paper that explores how distorted subjective expectations influence asset prices. The authors present the Subjective Belief Factor (SBF), linking subjective beliefs with excess returns, offering a fresh way to integrate beliefs and preferences in asset pricing.
Today’s Sponsor
The 32,481% Boom: First Disruption to $martphones in 15 Years
Mode saw 32,481% revenue growth from 2019 to 2022, ranking them the #1 overall software company, on this year's Deloitte 500 fastest-growing companies list. Mode is on a mission to disrupt the entire industry with their “EarnPhone”, a budget smartphone that’s helped consumers earn and save $325M for activities like listening to music, playing games, and… even charging their devices?!
Mode has over $60M in revenue - this is your chance to invest in a $1T+ market opportunity!
This is a paid advertisement for Mode Mobile Reg A offering. Please read the offering statement at https://invest.modemobile.com/.
“Machine Learning and the Yield Curve: Tree-Based Macroeconomic Regime Switching”
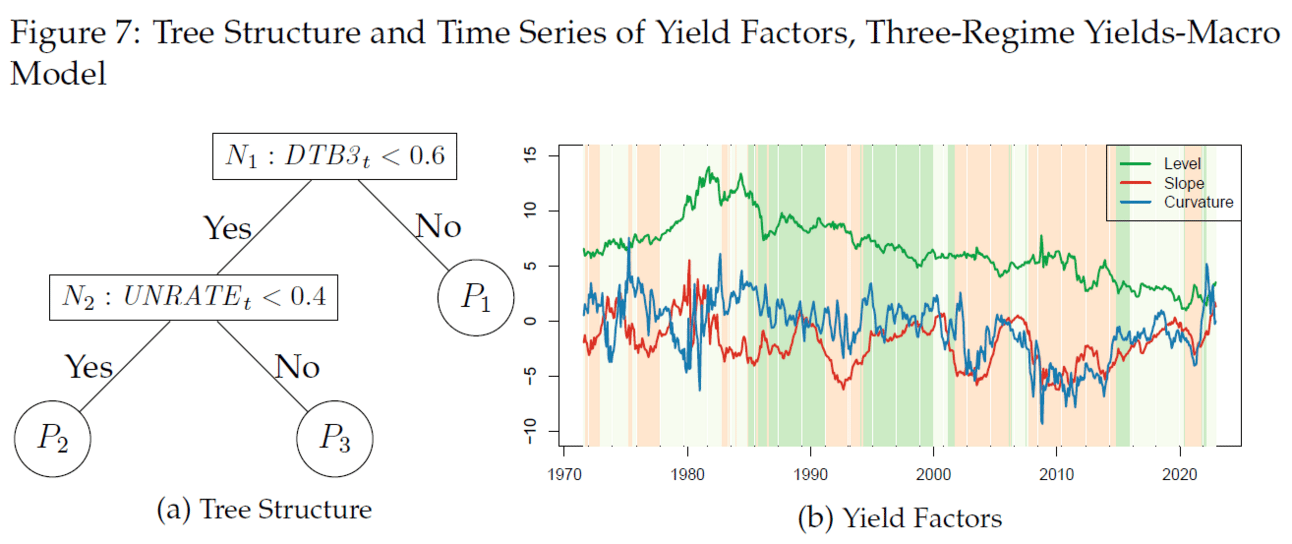
💊 New Investment Ideas! "Tree-Based Macroeconomic Regime Switching" How ML helps with yield curve prediction by uncovering hidden macroeconomic regimes.
Keep reading! 🔻
The paper explores a novel approach by applying machine learning to identify regime shifts in yield curves through a customized tree-based methodology, advancing beyond traditional Markov-switching models.
The main contributions and findings are as follows:
👉 The authors develop a dynamic Nelson-Siegel (DNS) yield-curve model integrated with a macroeconomic regime-switching framework using decision trees. This model uncovers regime shifts driven by macroeconomic conditions, offering a more interpretable structure compared to latent, opaque regimes in traditional models.
👉 By applying this model to U.S. Treasury bond yields, the paper demonstrates significant regime switching linked to the macroeconomic environment, revealing that certain macro variables predict yield curve movements, particularly when short-term rates are high.
👉 The method introduces computational simplicity while preserving the complexity of macro-financial linkages, outperforming traditional approaches by providing clear economic interpretations of regime changes.
👉 Bayesian estimation techniques ensure robust performance in identifying optimal regimes and transitions, enhancing yield prediction accuracy across different macroeconomic conditions.
👉 This macro-instrumented regime-switching model is versatile and can be extended to address broader macro-financial relationships, suggesting a regime-specific validity of key asset pricing theories such as macro-spanning.
“Optimal Factor Timing in a High-Dimensional Setting”

The paper presents a disciplined approach to factor timing in equity portfolios using shrinkage techniques to improve out-of-sample performance.
The main contributions and findings are as follows:
👉 The authors propose a framework for timing equity factors, leveraging machine learning to combine numerous predictors, including macroeconomic variables and factor-specific characteristics.
👉 The method applies shrinkage, which tempers expectations of extreme gains, preventing over-optimization while improving portfolio stability.
👉 Tested on Fama-French factors and large-cap factors, the framework boosts performance, delivering higher Sharpe ratios than equal-weighted or static strategies.
👉 The method is scalable, performing well even with thousands of factor-predictor combinations, and includes a high-dimensional shrinkage approach similar to ridge regression.
👉 Despite the complexity, the model generates intuitive results, such as adjusting exposure based on macro variables like the yield curve, enhancing predictability in factor returns.
Sponsor 👇
Maximize Your Portfolio with These Free Daily Trade Alerts
Master the market in just 5 minutes per day
Hot stock alerts sent directly to your phone
150,000+ active subscribers and growing fast!
AI-Essentials
In this video, I introduce a tool that is a must for every developer. I've recently been experimenting with Cursor AI, and it’s a game changer. Even if you're not a full-time developer, like me, you have to give it a try.
Asset Pricing Insights
“The Subjective Belief Factor”

This paper introduces a Subjective Belief Factor (SBF) that quantifies how distorted subjective expectations affect asset prices. By linking subjective beliefs with excess returns, the authors provide a novel way to integrate beliefs and preferences in asset pricing.
Key contributions and findings include:
👉 The SBF is a distortion variable that helps explain differences between subjective expectations and statistical forecasts for macroeconomic variables like real GDP growth and the T-bill rate.
👉 Applying the SBF to cross-sectional stock returns shows that it explains a large portion of excess returns, including Fama-French factors and numerous stock market anomalies.
👉 The framework helps distinguish whether asset price anomalies arise from biased beliefs or risk-based preferences, offering a clearer understanding of the drivers of asset prices.
👉 By summarizing expectations with a single SBF, the method simplifies analysis across many variables and performs well, even in high-dimensional settings, akin to modern machine learning techniques.
If you're enjoying our newsletter and want to support us, please recommend it to anyone you know who's interested in AI and Finance. Your referrals are the biggest compliment and help us grow! 🌟🤖💼