- The AI Finance Frontier
- Posts
- Machine Learning for Short Straddles on the S&P500
Machine Learning for Short Straddles on the S&P500
Supervised Machine Learning Classification for Short Straddles on the S&P50
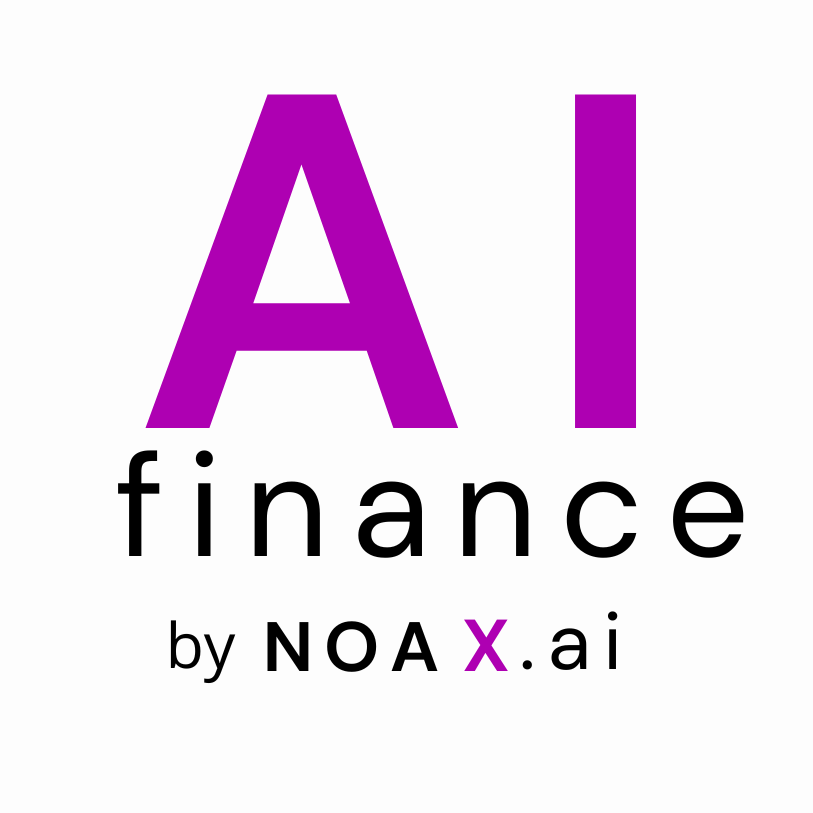
Hi! Here's Iván with this week's exciting newsletter, brimming with insights and discoveries on building robust investment strategies and risk models using Machine Learning.
In this edition, I am presenting the following sections:
🕹️ AI-Finance Insights: I summarize three must-read academic papers that mix cutting-edge ML/DL with Quant Finance:
Supervised Machine Learning Classification for Short Straddles on the S&P500
The Virtue of Complexity in Return Prediction.
Conditional GAN for Portfolio-Based Investment Strategies.
💊 AI Essentials: The section on top AI & Quant Finance learning resources: Today, I introduce a GitHub repository with 150 scripts designed for quantitative finance, algorithmic trading, and market data analysis.
🥐 Quant Finance Insights: In this edition, I provide a brief overview of how to improve your Alpha by combining factors (anomalies) using ML/DL.
Today’s Sponsor
The Wine Club Supporting Independent Winemakers
Naked Wines connects you directly to the world’s best indie winemakers. As America’s largest DTC winery, Naked Wines strips the hassle and extra costs out of wine delivery to serve you top-quality wines for up to 60% less. Take a short quiz, select your preferred quantity of wines, and Naked Wines does the rest to provide you with regular shipments of world-class wines. Customers only pay for wines they love - no questions asked.
AI-Finance Insights
“Supervised Machine Learning Classification for Short Straddles on the S&P500”

This is a simple application of meta-labeling in options strategies that can serve as a source of new ideas; discussing the potential application of meta-labeling to options strategies is worthwhile.
In my opinion, one of the most effective uses of ML/DL is to provide additional "incremental" improvement on top of existing algorithmic strategies.
This paper serves as an example. Briefly, the authors employ a supervised approach to assign a probability to the success of well-known straddle strategies on a daily basis.
Here, I summarize the work in a nutshell: 👇
➡ The study introduces machine learning models to implement specific short-option strategies on the S&P 500, focusing on a supervised classification task to assess the daily viability of executing short straddles.
➡ The methodology and evaluation criteria for different classification models are detailed, showcasing a systematic approach to hyperparameter optimization.
➡ The research identifies a statistically significant benefit when employing the gradient tree boosting algorithm over a simplistic "trade always" strategy, indicating a strategic edge in certain conditions.
➡ This research represents a first step for applying supervised classification methods to broader derivative trading strategies, marking a significant step forward in refining machine learning applications for financial markets.
“The Virtue of Complexity in Return Prediction”

The paper provides a theoretical analysis rationalizing why ML/DL models (or "complex" models) outperform simple models in the prediction of stock returns.
Let me summarize the paper in just 4 key takeaways: 👇
➡ The study challenges the effectiveness of "simple" models, which use only a few parameters for predicting market returns, demonstrating theoretically that these models significantly understate return predictability compared to "complex" models, where parameters outnumber observations.
➡ Through empirical evidence, the virtue of complexity in predicting U.S. equity market returns is documented, underscoring the superiority of complex models over traditional simple ones.
➡ The findings advocate for the use of machine learning in modeling expected returns, highlighting its potential in capturing nuances that simple models overlook.
➡ This research sets a precedent for integrating machine learning into financial market predictions, emphasizing the shift towards more sophisticated, data-driven approaches for enhanced accuracy.
“Conditional GAN for Portfolio-Based Investment Strategies”

A new approach to portfolio allocation utilizes GANs, introducing a framework based on conditional GAN (CGAN) that incorporates a mechanism for normalizing data using projected mean values of future series, leading to stable strategies. This is called "HybridCGAN".
Here, I summarize the paper in a nutshell: 👇
➡ The paper introduces a novel hybrid conditional Generative Adversarial Network (GAN) strategy for portfolio analysis, enhancing traditional methods by predicting market trends alongside managing uncertainties.
➡ It critiques the Markowitz framework and existing GAN approaches for their focus on series generation and trend identification rather than future forecasting.
➡ By integrating deep generative models, the proposed HybridCGAN and HybridACGAN demonstrate superior portfolio allocation against conventional Markowitz, CGAN, and ACGAN methods, validated through datasets from US and European markets.
➡ This advancement highlights the potential of advanced machine learning techniques in improving market trend prediction and portfolio optimization, marking a significant development in financial market analysis and derivative trading strategy innovation.
AI-Essentials
Discover a comprehensive GitHub repository featuring over 150 scripts tailored for quantitative finance, algorithmic trading, and market data analysis. This repository serves as a prime resource for collecting, processing, and examining stock market data. 👇
Quant Finance Insights
“The Expected Returns on Machine-Learning Strategies”
How to improve your Alpha by combining factors (anomalies) using ML/DL?
I summarize a nice piece of research with many interesting takeaways for ML/DL-based investors in less than 2 min 👇
➡ The study evaluates machine learning-based trading strategies with anomalies (factors) as inputs, considering transaction costs and liquidity changes.
➡ The paper utilizes a comprehensive dataset that includes signals based on anomalies, macro-indicators, and industry dummies, resulting in 376 features per observation for their models.
➡ Sophisticated machine learning strategies can profit, with monthly returns up to 1.42%, despite high turnover.
➡ A strategy using a long-short-term memory model shows a net alpha of 1.20%.
➡ Cost reduction techniques don't enhance performance.
➡ Deep-learning models predict returns beyond common risk factors, showing unexplained profitability.
If you're enjoying our newsletter and want to support us, please recommend it to anyone you know who's interested in AI and Finance. Your referrals are the biggest compliment and help us grow! 🌟🤖💼