- The AI Finance Frontier
- Posts
- Maximally Machine-Learnable Portfolios
Maximally Machine-Learnable Portfolios
How to maximize predictability in portfolio construction?

Hi! Here's Iván with this week's exciting newsletter, brimming with insights and discoveries on building robust investment strategies and risk models using Machine Learning.
In this edition, I am presenting the following sections:
🕹️ AI-Finance Insights: I summarize three must-read academic papers that mix cutting-edge ML/DL with Quant Finance:
Maximally Machine-Learnable Portfolios
Bloated Disclosures: Can ChatGPT Help Investors Process Information?
Pairs Trading Using Clustering And Deep Reinforcement Learning
💊 AI Essentials: The section on top AI & Quant Finance learning resources: Today, I'm thrilled to present a video that delves deeply into the mathematical foundations of Kolmogorov-Arnold Networks (KANs). This comprehensive guide explores the original paper that introduced these networks, providing detailed explanations of the key mathematical concepts involved.
🥐 Asset Pricing Insights: In this edition, I recommend timely research that investigates the real drivers behind value investment performance. A must-read to understand factor timing.
Today’s Sponsor
Ready to revolutionize your workday with AI?
Discover the key to unlocking unparalleled productivity with HubSpot’s free guide to using ChatGPT at work. You’ll find practical insights, useful integrations, and 100 prompt ideas to help you unleash the power of AI for a more efficient, impactful professional life.
AI-Finance Insights
“Maximally Machine-Learnable Portfolios”
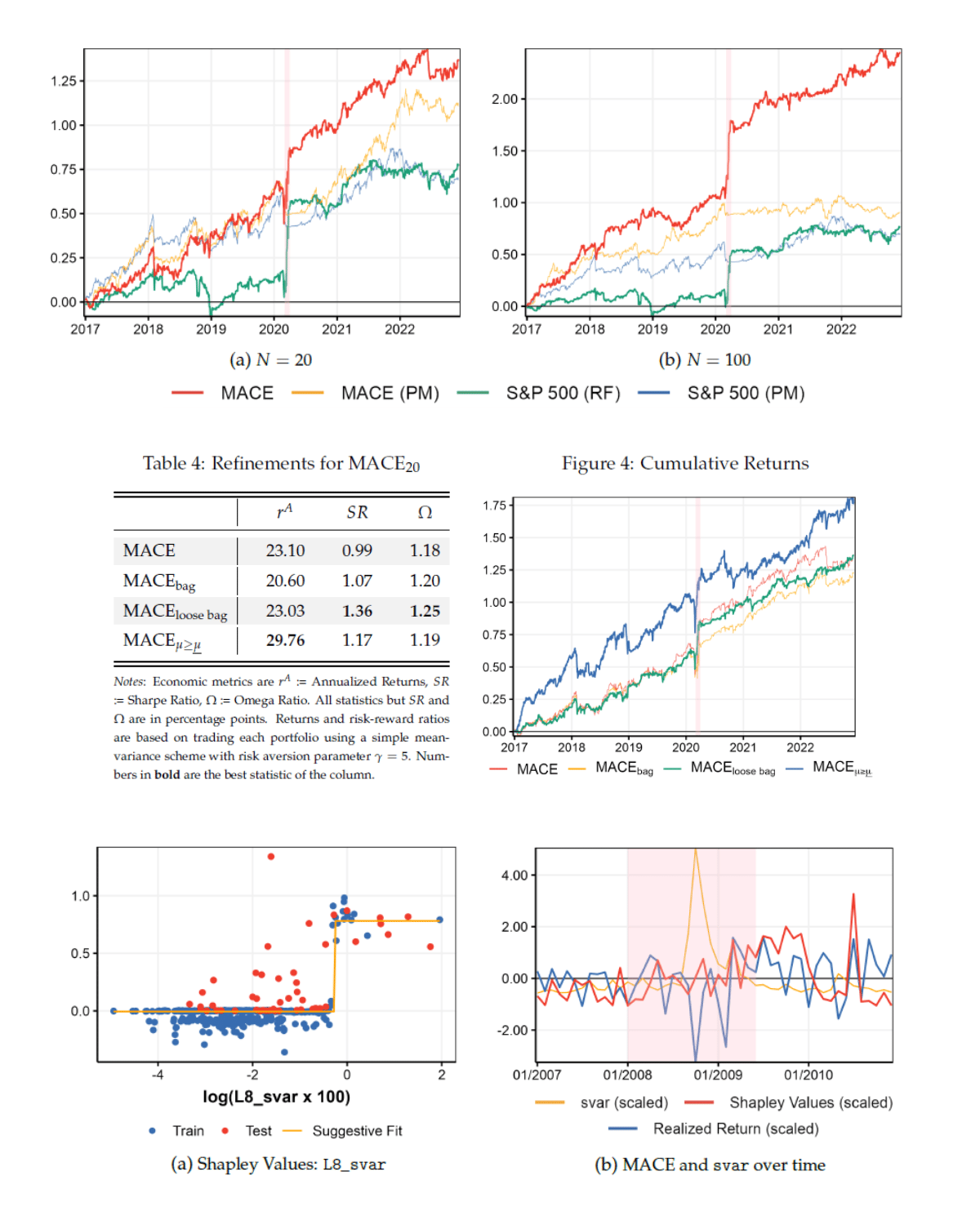
How to maximize predictability in portfolio construction? 👇
The paper introduces a collaborative machine learning algorithm that optimizes portfolio weights to create synthetic securities with maximum predictability.
Here are the key insights from the paper:
🔎 The paper presents MACE, an advanced version of Alternating Conditional Expectations, designed to optimize portfolio weights for maximum predictability. The model leverages a Random Forest and constrained Ridge Regression to achieve its goals.
🔎MACE improves earlier models by accommodating any forecasting algorithm and predictor set while handling large portfolios. It improves predictability and profitability even during adverse market conditions like the 2022 downturn.
🔎The first application evaluated daily portfolios of 20, 50, and 100 large NASDAQ firms from 2017 to 2022, showing notable returns and strong risk-reward profiles by employing mean-reverting strategies.
🔎The second application focused on monthly frequency, using macroeconomic indicators and large-cap stocks. MACE achieved substantial predictability improvements, exceeding 4% R2 over the past 15 years. The model effectively managed risk during crises and adjusted to market volatility.
“Bloated Disclosures: Can ChatGPT Help Investors Process Information?”

How can generative AI tools like ChatGPT fundamentally transform the way investors process information? The paper tries to answer this question.
Here are the key insights from the paper ⤵
🏷 The paper examines the utility of generative AI, like ChatGPT, for investors processing complex corporate disclosures. Using the stock market as a testing ground, the study shows that AI-generated summaries of MD&A and earnings conference calls can significantly condense the information, producing summaries that are just 25% to 30% of the original documents' length.
🏷 Despite omitting many details, the AI summaries still retain key information. They reflect the sentiment of the original documents, becoming more positive or negative accordingly. This effectively explains how stock market reactions align with the disclosed information.
🏷 The study introduces a measure of "information bloat," showing that overly complex disclosures correlate with reduced price efficiency and increased information asymmetry.
🏷 The model, GPT-3.5 (I know it is already "old"), effectively summarizes documents without external references while also specifically targeting financial and ESG-related information. This enhances the ability to identify firms' financial and non-financial performance.
🏷 Overall, generative AI significantly helps investors with information processing constraints, improving their understanding and reaction to corporate disclosures.
“Pairs Trading Using Clustering And Deep Reinforcement Learning”

Nice source for new ideas to improve classical pairs trading/statistical arb strategies.
The paper in a nutshell:
✴ The paper investigates enhancements to traditional pairs trading strategies, which typically rely on linear, mean-reverting relationships, by integrating deep learning to address non-linear dynamics in equity markets.
✴This involves a novel two-step methodology: clustering equity indices using latent risk factors identified by a convolutional auto-encoder, followed by deploying a Reinforcement Learning-based trading strategy on these clusters.
✴The evaluation of this methodology was conducted using 13 pairs of indices from April 2017 to December 2022. The results highlight the NASDAQ Composite and MSCI World IT Index pair as the standout performer, achieving an annualized return of 21.86% and a Sharpe ratio of 1.15, substantially surpassing the S&P 500 benchmark.
✴Comprehensive analysis shows all sampled pairs outperformed the benchmark, with the top three pairs registering annualized returns of 21.86%, 18.61%, and 17.61% respectively, illustrating the effectiveness of the trading strategy in leveraging non-linear relationships.
✴The strategy demonstrated key attributes of statistical arbitrage, notably market-neutrality, which enabled it to maintain stable returns even during periods of significant market volatility, such as during the COVID-19 market downturn in March 2020.
AI-Essentials
Have you heard about KANs ("Kolmogorov-Arnold Networks")? The following video explains, going deep into the mathematical details when needed, the original paper that introduced these networks. 👇
Sponsor 👇
Become an AI & ChatGPT Genius in just 3 hours for FREE! (Early Easter Sale)
Join ChatGPT & AI Workshop (worth $199) at no cost (Offer valid for first 100 people only) 🎁
Asset Pricing Insights
“What Drives Booms and Busts in Value?”

Timely research on the real drivers of value investment performance. Keep reading! 👇
Value investing experiences high volatility and significant drawdowns across different market conditions. The paper explores these returns using the intertemporal CAPM (ICAPM), showing that aggregate cash flow, discount rate, and volatility significantly influence value returns, which aligns with findings over the past 60 years.
The study differentiates between intra-industry and inter-industry value components, revealing that the ICAPM better explains intra-industry movements.
Additionally, it highlights that value booms and busts don't align with overall market returns, as evidenced during periods like the global financial crisis and the Covid-19 pandemic. Instead, intra-industry returns primarily drive value fluctuations, which are analyzed through an intra-industry value strategy focusing on stocks with relatively high book-to-market ratios within their industry.
If you're enjoying our newsletter and want to support us, please recommend it to anyone you know who's interested in AI and Finance. Your referrals are the biggest compliment and help us grow! 🌟🤖💼