- The AI Finance Frontier
- Posts
- Pair-Trading Strategies using Machine Learning
Pair-Trading Strategies using Machine Learning
Explore and Uncover: In-Depth Ideas and Strategic Insights on Designing and Applying Pair-Trading Strategies Using Machine Learning


Hi! Here's Iván with this week's exciting newsletter, brimming with insights and discoveries on building robust investment and trading strategies using Machine Learning. This edition stands out for its focus on Pair-Trading Strategies using Machine Learning. We're highlighting:
🕹️ 2 Academic Articles: Dive into groundbreaking research featuring actionable ideas that are reshaping our understanding of how to apply ML/DL in creating successful investment and trading strategies.
💊 Video Insight : Simplified ML/DL Techniques for Pair-Trading and Investment.
✔️ Market Insight: Best market reflection shared on my LinkedIn/Twitter during the last week: the financial market is always first!
🥐 Methodological Insight for ML Investment Strategies: More advanced methodological topics to deepen your understanding of how to design profitable ML/DL trading strategies.
Academic Insights
“Pairs Trading via Unsupervised Learning”

Simple but powerful starting point to build a machine learning pair trading strategy. 👇
Summary of the paper in only 3 ideas:
The paper utilizes three unsupervised learning methods to construct pair trading stratagies: the classical k-means, agglomerate clustering or DBSCAN method.
Comparing the performance of the long-short portfolios constructed by the three clustering methods, they find that the agglomerative clustering significantly outperforms the others, attaining an annualised mean return of 24.8%, an annualised Sharpe ratio of 2.69, and a low maximum drawdown of 12.3%, which lasts only for two months.
The feature set consists of 48 return factors and 78 firm characteristics generated every month for the sample period from December 1979 to November 2020. The out-of-sample period is from January 1980 to December 2020.
“Mastering Pair Trading with Risk-Aware Recurrent Reinforcement Learning”
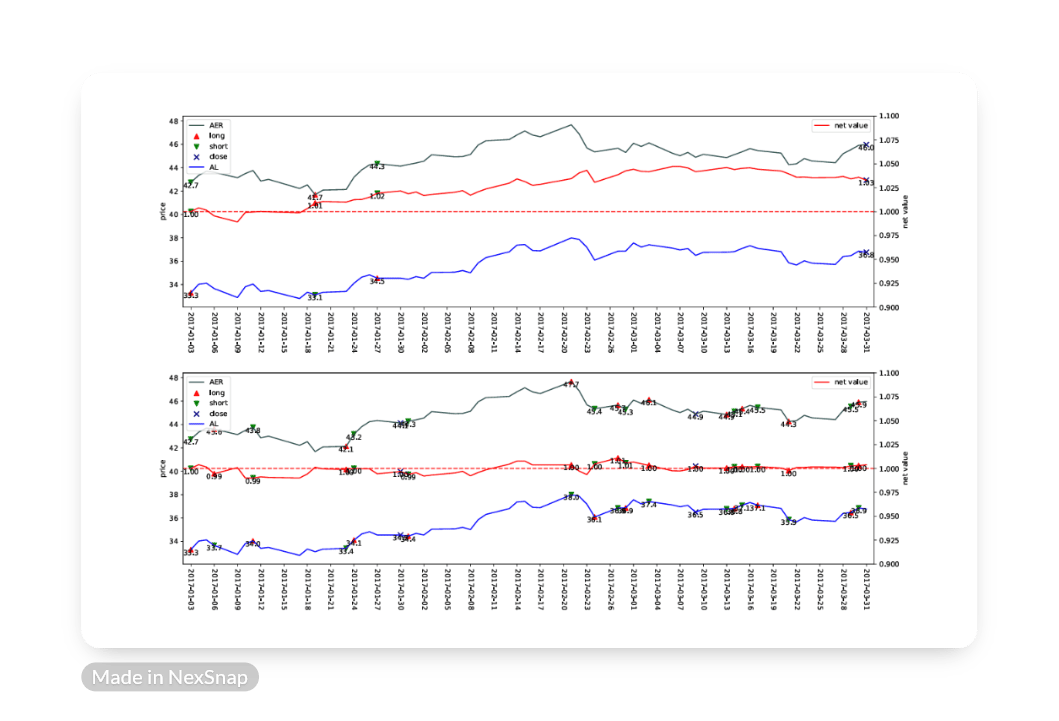
Summary of the paper in only 5 ideas:
Existing traditional RL methods ignore the temporal relationship between asset price movements and the risks associated with certain trades. This leads to over-trading, thereby increasing the transaction costs and potential losses of the strategies.
In the paper, the authors introduce a risk-aware agent capable of learning long-term opportunities, mimicking human pair trading experience.
The model is the first to apply a bidirectional GRU along with the temporal attention mechanism to fully consider the temporal correlations embedded in the states.
They also design the risk-aware reward, inspired by economic theory, that models both the profit and risk of the trades during the trading period.
Experiments show that it outperforms existing reinforcement learning methods in pair trading and achieves a significant profit over five years of U.S. stock data.
AI-Essentials: Step-by-Step Tutorial
🚀 In today's newsletter, we're excited to share a video with a nice example about how to apply ML for pair-trading.
The post: Market Ideas
December through February period typically favors small caps over large caps. 👇

ML Investment Strategies: Methodological Insight

Can you "overfit" even out-of-sample? Yes, overfitting can occur out-of-sample too. How can it be mitigated? 👇
1. With large financial datasets, machine learning, and high-performance computing, analysts can test millions (if not billions) of alternative investment strategies. In ML/DL, this relates to the concept of "experimentation" in model development.
2. Moreover, backtest optimizers, also connected with hyperparameter tuning in ML, search for parameter combinations that maximize the simulated historical performance of a strategy/model.
3. The problem of performance inflation extends beyond backtesting. More generally, researchers and investment managers tend to report only positive outcomes, a phenomenon known as selection bias, which mainly affects out-of-sample results.
4. Not controlling for the number of trials involved in a particular discovery leads to overly optimistic performance expectations. A potential solution, albeit relatively 'old' and still underutilized in many papers and industry applications, is the "Deflated Sharpe Ratio (DSR)".
5. DSR corrects for two leading sources of performance inflation: (i) Selection bias under multiple testing and (ii) non-normally distributed returns. In doing so, DSR helps separate legitimate empirical findings from statistical flukes.
If you're enjoying our newsletter and want to support us, please recommend it to anyone you know who's interested in AI and Finance. Your referrals are the biggest compliment and help us grow! 🌟🤖💼