- The AI Finance Frontier
- Posts
- Predicting the VIX with Computer Vision
Predicting the VIX with Computer Vision
Images from historical equity options surface data to predict the VIX index
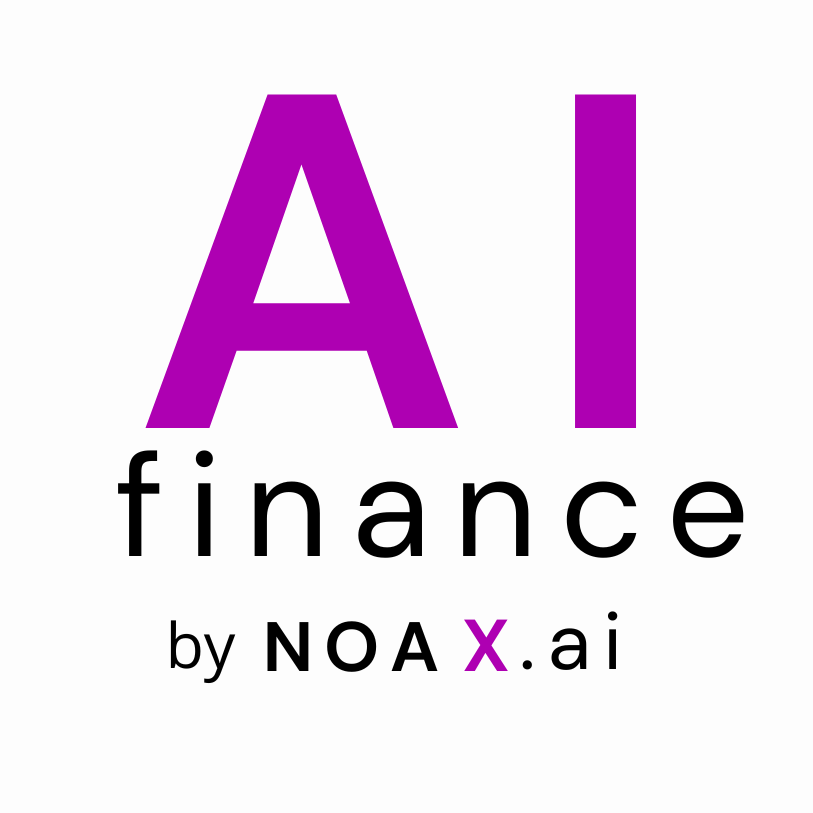
Hi! Here's Iván with this week's exciting newsletter, brimming with insights and discoveries on building robust investment strategies and risk models using Machine Learning.
In this edition, I am presenting the following sections:
🕹️ AI-Finance Insights: I summarize three must-read academic papers that mix cutting-edge ML/DL with Quant Finance:
Predicting the VIX with Computer Vision.
Satellite Images for Stock Prediction.
Deep Learning Two-Stage ETF Sector Rotation.
💊 AI Essentials: The section on top AI & Quant Finance learning resources. Today, I present 5 essential books for starting with machine learning, statistics, and quant finance.
🥐 Quant Finance Insights: In this edition, I provide a brief overview of how to mitigate the risk of test sample overfitting when backtesting your strategies.
Today’s Sponsor
Are you ready to take your investing game to the next level?
We empower investors like you with a daily newsletter which includes the insights needed to stay informed and make profitable decisions in the stock market.
Join Millions Who Trust The Early Bird
Discover daily recommendations
Unlock hidden opportunities
Make informed investment decisions
By receiving our #1 stock tip delivered straight to your inbox every day at 6:59 am—completely FREE.
Seize the Opportunity:
Join the ranks of millions of investors who trust The Early Bird. Sign up now to receive today's #1 Trade of the Day and unlock a world of exclusive benefits.
Your success in the market is just one click away!
AI-Finance Insights
“Predicting the VIX with Computer Vision”

The paper utilizes images from historical equity options surface data to predict future VIX index levels.
I summarize the paper in just 5 takeaways 👇
➡ The research investigates the potential of CNN, CONV-LSTM, and Transformer models for volatility prediction, which are not yet standard in the industry.
➡ Historical volatility surface data from OptionMetrics on the S&P 500 Index is processed into grayscale images to feed into the models, targeting VIX index values 1, 5, and 10 days into the future.
➡ The study benchmarks these innovative approaches against traditional models like linear regression and GARCH, showing superior predictive power in the advanced methods.
➡ Findings reveal that CONV-LSTM and Transformer models significantly outperform other techniques, indicating a new direction for volatility forecasting.
➡ The integration of machine learning and deep learning into volatility prediction exemplifies their effectiveness beyond traditional financial models, offering tangible benefits for investors seeking to leverage ML/DL insights.
“Satellite Images for Stock Prediction”

What about using satellite images to predict stock indices based on estimations of container coverage? A thought-provoking idea that deserves a read.
Here's a simplified summary in less than 2 min: 👇
➡ The study introduces satellite imagery of shipping containers in ports as a real-time indicator of economic activity, overcoming traditional data's lag and revision issues.
➡ By analyzing 83,672 satellite images with the U-Net method, it quantifies container coverage to predict stock returns, using forecast combination over univariate predictive regression for accuracy.
➡ Results indicate significant prediction of stock index returns in 27 out of 33 countries, with an average annualized return of 16.38% for the 2019–2021 period, highlighting the method's profitability and reliability.
➡ The predictive power stems from the correlation between container numbers and economic activity, suggesting potential for broader economic forecasting applications.
➡ This research showcases satellite imagery as a potent tool for investors aiming for innovative and effective strategies in financial markets.
“Deep Learning Two-Stage ETF Sector Rotation”

An "easy-to-replicate" investment strategy based on sector ETFs, appropriate for mid/long-term investment.
The paper proposes a simple two-step process: 👇
➡ (Step 1) Using macroeconomic indicators and Recursive Feature Elimination (RFE), they select the indicators that are most important for each sector. The macro indicators come from the FRED website and Macrotrends. You can find the complete list in the appendix of the paper.
➡(Step 2) Using the selected indicators from step (1), they implement various Recurrent Neural Networks (RNN) to predict future ETF prices for each sector. They rank sectors based on the predicted returns, selecting the top 4 sector ETFs.
✒ The dataset comprises daily adjusted close prices for sector ETFs from July 14, 2000, to November 10, 2019, resulting in 4,862 prices per ETF, aligned with the NYSE market calendar.
✒ They use yfinance Python library for data download, our analysis primarily focuses on monthly data, yielding 233 monthly adjusted close prices.
✒They also conduct multiple robustness tests, adjusting the lookback window and the lookahead period.
✒ They compare the performance against an equally weighted portfolio, which, in my opinion, is not a very challenging benchmark.
Interestingly, they demonstrate that Echo State Networks (ESN) outperform other models.
AI-Essentials
Five essential resources for starting with machine learning, statistics, and quant finance. Take a look at them! 👇
"The Elements of Statistical Learning" https://buff.ly/3R14eIV
"All of Statistics" https://buff.ly/3Em0HgI
"Advances in Financial ML" (Lopez de Prado) https://buff.ly/2G9cjDV
"Linear Algebra Done Right" https://buff.ly/3sAHJAh
"(Free!) Deep Reinforcement Learning" https://buff.ly/3YYt4v3
Quant Finance Insights
“Mitigating Testing Set Overfitting”

Is winning the lottery with a "secret strategy" just luck if you buy enough tickets? Learn how this analogy reveals the issue of test overfitting in investment strategies! 👇
This situation mirrors how researchers might encounter false discoveries by running numerous statistical tests on the same data set.
Repeated testing increases the chance of an accidental find, similar to how fitting a model too closely to the test set, rather than the training set, can lead to misleading results.
🔔 Solutions to mitigate this concern:
➡ The Deflated Sharpe Ratio adjusts for the number of attempts, similar to accounting for the number of lottery tickets bought by your friend.
➡ Increasing the number of test sets makes it harder to overfit across thousands of them.
➡ Monte Carlo methods generate synthetic data that mimic the behavior and statistical properties of the underlying series, requiring a robust data-generating process (where ML/DL can be highly effective). The primary benefit of this approach is that conclusions are drawn from a distribution of random realizations rather than a single observed instance of the data-generating process. Analogous to the lottery example, it's like simulating the lottery multiple times to eliminate the influence of luck.
References: "Machine Learning for Asset Managers" (Marcos M. López de Prado)
If you're enjoying our newsletter and want to support us, please recommend it to anyone you know who's interested in AI and Finance. Your referrals are the biggest compliment and help us grow! 🌟🤖💼