- The AI Finance Frontier
- Posts
- Statistical Arbitrage in Rank Space
Statistical Arbitrage in Rank Space
Portfolios achieving an impressive annualized return of 35.68% and a Sharpe ratio of 3.28.

Hi! Here's Iván from Noax Capital with this week's exciting newsletter, brimming with insights and discoveries on building robust investment strategies and risk models using Machine Learning.
In this edition, I am presenting the following sections:
🕹️ AI-Finance Insights: I summarize two must-read academic papers that mix cutting-edge ML/DL with Asset Pricing & Quant Finance:
Statistical Arbitrage in Rank Space
Heteroskedastic SDF and Learning about Time-Varying Factor Risk Premia
💊 AI Essentials: The section on top AI & Quant Finance learning resources: Today, I’m sharing a practical video titled “Machine Learning with Python and Scikit-Learn: A Beginner’s Guide.” This course offers a hands-on introduction to machine learning fundamentals, perfect for those with a basic background in Python and statistics. If you're eager to dive into the world of AI and data-driven insights, this is an excellent place to start!
🥐 Asset Pricing Insights: In this edition, I introduce a paper titled “Dynamic Factor Allocation Leveraging Regime-Switching Signals.” This study presents a pioneering approach to factor allocation by incorporating regime-switching signals to better capture the cyclic performance of factors.
“Statistical Arbitrage in Rank Space”
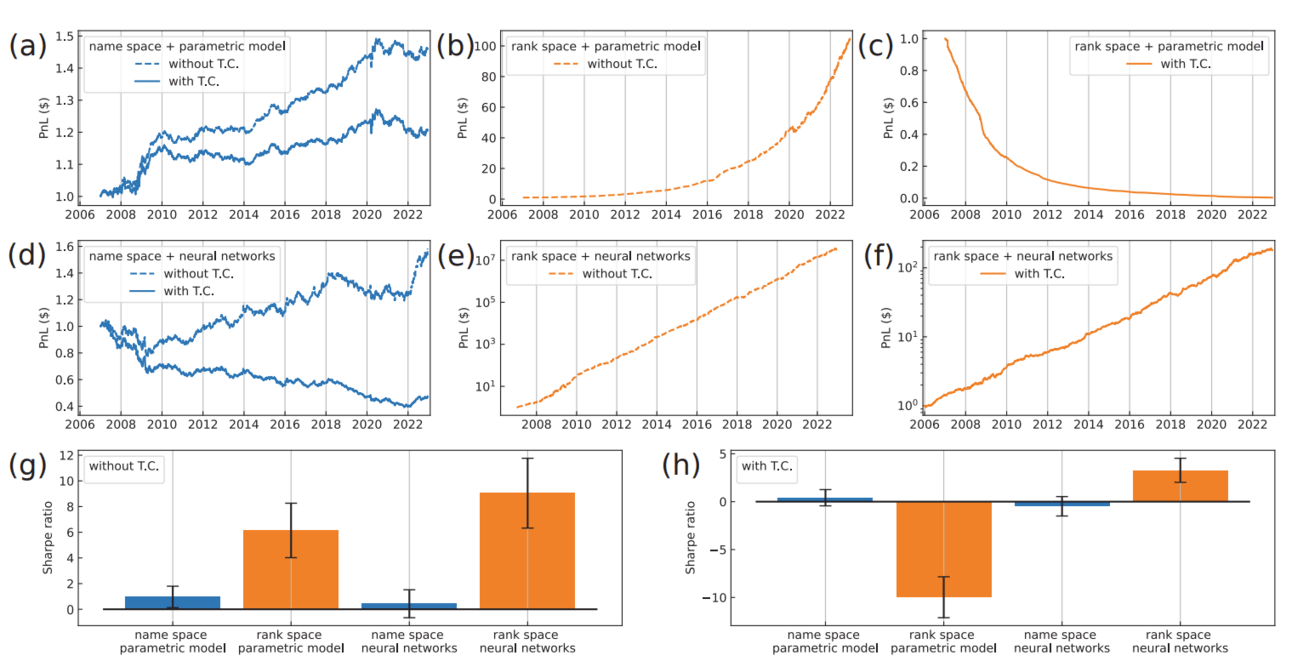
👉 This paper introduces a “rank space” approach, where stocks are indexed by capitalization ranks instead of names, leading to more stable market structures and a new perspective on equity dynamics.
👉 Enhanced mean-reversion properties in rank space allow for superior statistical arbitrage, with portfolios achieving an impressive annualized return of 35.68% and a Sharpe ratio of 3.28 over the 2007–2022 period.
👉 An intraday rebalancing mechanism maintains market neutrality by dynamically adjusting to real-time rank changes, strengthening robustness against market noise and delivering optimized trading signals.
👉 Backtesting results show that rank-space strategies, particularly when neural networks are employed, significantly outperform traditional methods, even with transaction costs, underscoring their potential in high-dimensional portfolios.
“Heteroskedastic SDF and Learning about Time-Varying Factor Risk Premia”

👉 This paper explores the volatility and conditional heteroskedasticity of the stochastic discount factor (SDF) by modeling time-varying factor risk premia and factor covariance. The authors introduce a Bayesian learning approach, enabling a real-time belief update on model parameters, which contributes to the conditional heteroskedasticity of the SDF.
👉 Using the Fama-French six factors, the study finds strong evidence of factor timing driven by factor momentum and macro predictors, as well as volatility timing. This dynamic approach demonstrates high forecasting power for risk premia, particularly for certain factors such as HML and RMW, significantly outperforming traditional models.
👉 Key results: The Bayesian learning model, incorporating both factor and volatility timing, achieves an annualized Sharpe ratio of up to 1.40, showcasing substantial predictive accuracy and superior performance post-2008 financial crisis compared to classic pricing models.
👉 This model is particularly effective in volatile markets, enhancing real-time adaptability and capturing market dynamics that traditional models may miss. The findings highlight the practical benefits of Bayesian learning and adaptive volatility management in constructing optimal portfolios.
AI-Essentials
IIn this edition, we're featuring an exciting introductory course on Machine Learning with Python and Scikit-Learn, designed for beginners with a basic understanding of Python and statistics. This hands-on course provides practical skills to kickstart your journey in machine learning, guiding you through essential concepts, tools, and techniques to build and evaluate models effectively.
Asset Pricing Insights
“Dynamic Factor Allocation Leveraging Regime-Switching Signals.”

👉 This paper presents a pioneering approach to factor allocation by incorporating regime-switching signals to better capture factor performance cycles. The authors use a sparse jump model (SJM) to dynamically classify market regimes for factors, helping investors adjust allocations based on bull or bear conditions specific to each factor.
👉 Unlike traditional timing strategies, this method emphasizes identifying current regimes rather than predicting shifts, aiming to optimize factor exposure as cycles evolve. The integration of these signals with the Black-Litterman model results in a robust multi-factor portfolio that dynamically shifts allocations among value, size, momentum, quality, low volatility, and growth factors.
👉 This dynamic approach achieves notable improvements in risk-adjusted returns, with an impressive Sharpe ratio of 0.65 compared to 0.54 for the equally weighted benchmark, and an information ratio of 0.4 to 0.5 relative to the benchmark. Additionally, the strategy achieves a 12.5% annualized excess return with a maximum drawdown reduction to −50.5% (from −54.9% for the market).
👉 By leveraging regime persistence, the model enhances factor allocations, especially in complex environments. This research offers valuable insights for investors seeking more adaptive, responsive factor-based strategies in diverse market conditions.
If you're enjoying our newsletter and want to support us, please recommend it to anyone you know who's interested in AI and Finance. Your referrals are the biggest compliment and help us grow! 🌟🤖💼