- The AI Finance Frontier
- Posts
- Statistical arbitrage powered by Explainable Artificial Intelligence
Statistical arbitrage powered by Explainable Artificial Intelligence
Discover the power of Explainable AI (XAI) in building robust investment strategies.
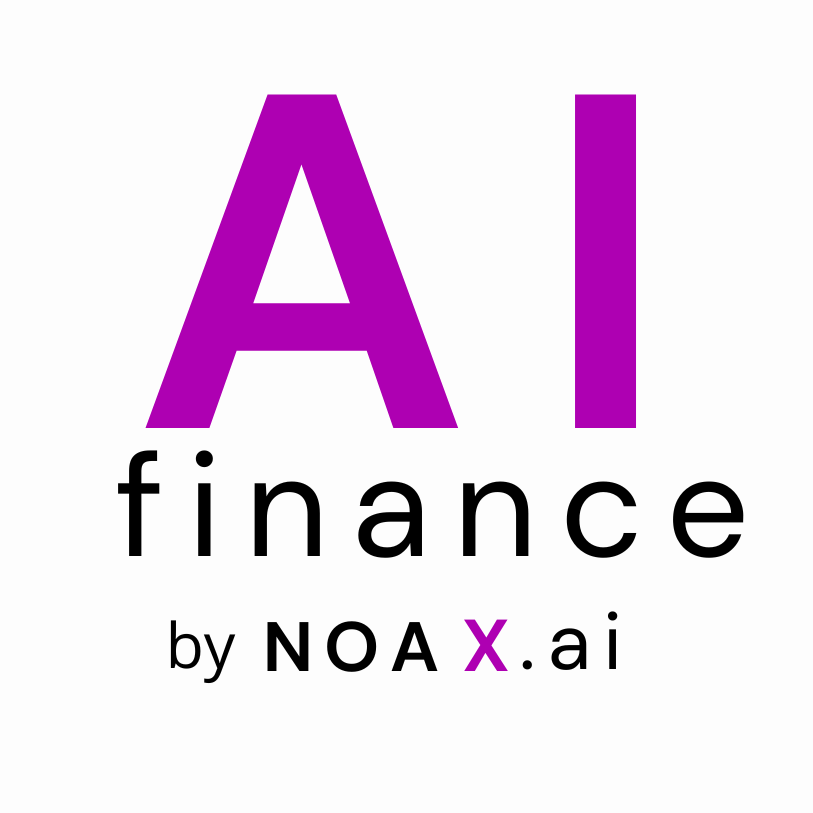
Hi! Here's Iván with this week's exciting newsletter, brimming with insights and discoveries on building robust investment strategies and risk models using Machine Learning.
In this edition, I am presenting the following sections:
🕹️ AI-Finance Insights: I summarize three must-read academic papers that mix cutting-edge ML/DL with Quant Finance:
Statistical arbitrage powered by Explainable Artificial Intelligence
Forecasting the Equity Premium: Mind the News!
Fundamental Analysis via Machine Learning
💊 AI Essentials: The section on top AI & Quant Finance learning resources: Today, I'm sharing 5 free AI courses released by NVIDIA, essential for anyone seeking a hands-on introduction to AI from various perspectives.
🥐 Sentiment Insights: In this edition, I recommend a paper exploring how sentiment indices enhance tactical asset allocation strategies.
Today’s Sponsor
How did @TheArbFather make $10,000+ profit in February?
His secret sauce: Arbitrage betting.
Instead of getting bettings tips from slick-talking handicappers, TheArbFather bets on both sides of an outcome to guarantee a risk-free return. An example of this is betting on a game total to be over 224.5 AND under 224.5.
This might sound too good to be true, but the reason this can happen is sportsbooks set their lines for games independently. Sometimes, they make mistakes and there are situations where FanDuel’s odds are different from DraftKings’ odds.
Unless you are a PhD-wielding, Python-coding, Excel-Wizard… finding arbitrage opportunities consistently has been out of reach. Until now…
OddsJam scans millions of odds every second and finds these need-in-a-haystack opportunities that you can bet on to secure a risk-free profit.
AI-Finance Insights
“Statistical arbitrage powered by Explainable Artificial Intelligence”

In an ongoing project with one of our consulting clients (an institutional investor seeking AI improvements for a daily frequency Long-Short strategy), we've experienced firsthand how beneficial it is for statistical arbs strats to reduce the noise-to-signal ratio by strategically selecting features leveraging XAI (eXplainable Artificial Intelligence tools).
This paper offers a similar approach and is worth the read. Here are my simplified takeaways from the paper: 👇
➡ This paper dives into the combination of machine learning and eXplainable Artificial Intelligence (XAI) within the financial markets, focusing on improving statistical arbitrage trading strategies. It tackles the challenge of converting data into actionable insights and the drawbacks of solely depending on machine learning for decisions in the financial sphere.
➡ It presents three methodologies for removing irrelevant features from prediction models, tested on historical data of S&P500 index stocks. The aim is to not only refine prediction accuracy for individual stocks but also for the entire set, thus improving portfolio performance by eliminating data noise.
➡ The trading strategies introduced, which utilize feature selection
powered by XAI, demonstrate superior performance in risk-return analysis over traditional methods. These strategies involve buying the highest-performing and selling the lowest-performing stocks daily, guided by a sorting criterion derived from machine learning models trained on varied features, including lagged returns and technical indicators.
➡ By adopting a detailed process of feature selection, either for each stock or within particular industry sectors, the paper underlines the necessity of customizing models to accommodate the heterogeneous nature of stock data. This detailed approach allows for the identification of the most pertinent features for different data subgroups, leading to more accurate and explainable predictions.
➡ In summary, the study showcases the effectiveness of merging machine learning with XAI in financial decision-making, proposing a more reliable and insightful methodology for statistical arbitrage that surpasses conventional trading strategies.
“Forecasting the Equity Premium: Mind the News!”

This paper presents a new approach to forecasting monthly equity premia using over 700,000 newspaper articles from The New York Times and The Washington Post between 1980 and 2018.
Here are my simplified takeaways from the paper: 👇
➡ Leveraging a vast dataset, the study introduces a data-adaptive switching method to transform diverse news topics into accurate stock return forecasts, highlighting a novel use of machine learning and natural language processing in financial analysis. This technique proves particularly adept at capturing valuable insights not found in traditional economic indicators.
➡ The approach demonstrates substantial out-of-sample forecasting improvements, evidenced by an R2 OOS of 6.52% and considerable utility gains for mean-variance investors. The study notably underscores the enhanced predictive power of geopolitical over economic news, especially in downturn markets.
➡ Employing advanced statistical models, including the correlated topic model (CTM), this research effectively quantifies the impact of media coverage on stock performance. By averaging topic proportions monthly, it provides a more nuanced predictor for the S&P 500 index's monthly excess returns, moving beyond conventional prediction methods.
➡ The paper's findings suggest significant economic and statistical gains from this methodology, with a spotlight on its utility during market downturns. This aligns with and extends existing literature on the countercyclical predictability of stock returns, proposing an innovative angle for financial market analysis.
➡ Overall, this research illustrates the potent application of combining machine learning with extensive news data analysis to enhance financial forecasting. It sets a new benchmark for integrating natural language processing in the development of more informed, data-driven trading strategies, significantly advancing the field of quantitative finance.
“Fundamental Analysis via Machine Learning”

ML/DL proves beneficial even for fundamentally based investment firms. The application of AI extends well beyond algorithmic investing, and it's only a matter of time before AI models assist discretionary investment firms in enhancing their analyses.
Here are my 3 main takeaways from the paper: 👇
➡ This study reveals machine learning, particularly nonlinear models, enhances corporate earnings forecasts significantly beyond traditional methods, revealing deeper financial insights.
➡ It uncovers vital, economically sensible relationships between historical data and future earnings, impacting stock returns and analyst forecast errors, with notable market performance differences.
➡ The findings challenge the traditional reliance on linear models and aggregated data, suggesting the untapped potential of detailed financial statement analysis for investors' decision-making.
AI-Essentials
NVIDIA now offers FREE online AI courses.
Check out these 5 essential courses: 👇
Unveiling Generative AI
What's covered:
Understanding the basics of Generative AI.
Exploring applications of Generative AI.
Navigating the challenges and prospects of Generative AI.
Building A Brain in 10 Minutes
What's covered:
How neural networks learn from data.
The mathematics behind neurons.
Augment your LLM Using Retrieval Augmented Generation
What's covered:
Introduction to Retrieval Augmented Generation.
Overview of the RAG retrieval method.
Exploring NVIDIA AI Foundations and RAG model components.
Implementing AI in Data Centers
What's covered:
Overview of AI applications, Machine Learning, Deep Learning, and their processes.
The significance of GPU architecture in AI.
Insights into deep learning frameworks and deployment strategies.
Accelerate Data Science Workflows with Zero Code Changes
What's covered:
Advantages of integrating CPU and GPU workflows.
Accelerating data management and machine learning tasks without altering code.
Achieving quicker processing speeds.
Sentiment Insights
“Relative Sentiment and Machine Learning for Tactical Asset Allocation”

This study explores how Sentix sentiment indices can guide asset allocation. By comparing the 6-month economic outlook of institutional vs. individual investors in the U.S., Europe, Japan, and Asia (excluding Japan), it uses these sentiment differences along with upcoming month's equity returns as data for various machine learning models.
The study reveals that these sentiment contrasts are powerful predictors for identifying top and bottom global equity markets, outperforming both individual sentiment indicators and price momentum as forecasting tools.
🔔 In simpler terms, stock returns tend to be lower (higher) when retail investors are more (less) optimistic than institutions, with machine learning confirming these trends across different regions.
Ready to elevate your investing journey to new heights?
Unlock the power of informed decision-making with our daily newsletter, tailored to empower investors like you. The Early Bird delivers crucial insights that keep you ahead in the stock market, ensuring you make profitable decisions. Sign up today.
If you're enjoying our newsletter and want to support us, please recommend it to anyone you know who's interested in AI and Finance. Your referrals are the biggest compliment and help us grow! 🌟🤖💼