- The AI Finance Frontier
- Posts
- A Swing Trading Strategy Based on STL Decomposition and Self-Attention Networks
A Swing Trading Strategy Based on STL Decomposition and Self-Attention Networks
Swing trading that integrates STL decomposition and self-attention networks.
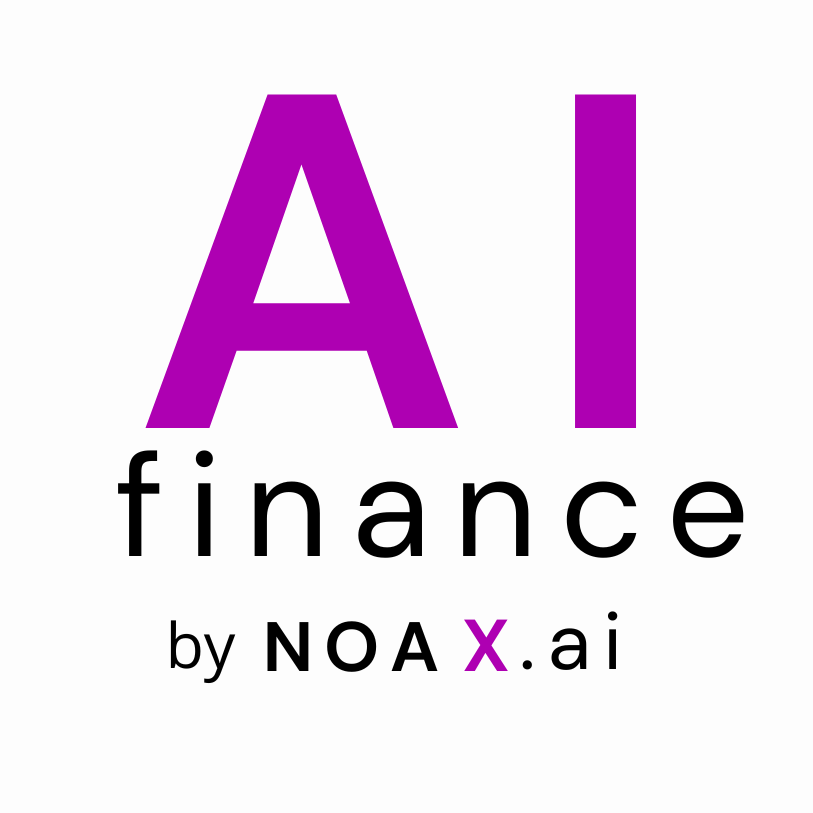
Hi! Here's Iván with this week's exciting newsletter, brimming with insights and discoveries on building robust investment strategies and risk models using Machine Learning.
In this edition, I am presenting the following sections:
🕹️ AI-Finance Insights: I summarize three must-read academic papers that mix cutting-edge ML/DL with Quant Finance:
A Swing Trading Strategy Based on STL Decomposition and Self-Attention Networks
Deep Learning in Asset Pricing
ChatGPT and Corporate Policies
💊 AI Essentials: The section on top AI & Quant Finance learning resources: Today, I'm sharing 5 free machine learning courses that are a must in 2024 if you want a hands-on introduction to machine learning from top institutions.
🥐 Macro Finance Insights: In this edition, I recommend a paper on macroeconomic forecasting and sovereign risk assessment using deep learning techniques.
Today’s Sponsor
The Rundown is the world’s fastest-growing AI newsletter, with over 500,000+ readers staying up-to-date with the latest AI news and learning how to apply it.
Our research team spends all day learning what’s new in AI, then distills the most important developments into one free email every morning.
AI-Finance Insights
“A Swing Trading Strategy Based on STL Decomposition and Self-Attention Networks”

Nice idea focusing on swing trading that integrates STL decomposition and self-attention networks.
Here are my 5 main takeaways from the paper: 👇
➡ In response to the need for sophisticated portfolio management tools amid market fluctuations and growing investor optimism, the paper introduces "Stockformer," an innovative deep learning framework optimized for swing trading, featuring the TopKDropout method for improved stock selection.
➡ By leveraging STL decomposition and self-attention networks, Stockformer excels in utilizing complex S&P 500 data, enhancing the accuracy of stock return predictions and offering a nuanced understanding of market dynamics.
➡ The framework's effectiveness is underscored during its test phase from February to June 2023, where Stockformer's ability to predict market trends outperforms ten leading industry models. It achieves a standout accuracy rate of 62.39% in detecting market trends, showcasing superior performance in key predictive accuracy indicators such as MAE, RMSE, and MAPE.
➡ Stockformer's application in swing trading strategies yields impressive financial results, generating a cumulative return of 13.19% and an annualized return of 30.80%. These figures significantly surpass those of current state-of-the-art models, indicating Stockformer's potential to revolutionize investment strategies.
➡ The paper concludes with a commitment to fostering innovation and collaboration within the financial technology community by open-sourcing Stockformer. This move not only underscores the framework's contribution to advancing market forecasting methodologies but also invites further development and application of Stockformer in the volatile investment landscape.
“Deep Learning in Asset Pricing”

The authors present a versatile asset pricing model accommodating a broad nonparametric form with numerous characteristics. This foundational model offers many intriguing investment insights—a must-read, in my opinion.
Here are my 5 main takeaways from the paper: 👇
➡ Utilizing deep neural networks, this study presents an asset pricing model for individual stock returns, which leverages extensive conditioning
information while maintaining flexibility and acknowledging time variations.
➡ Innovatively, it applies a no-arbitrage condition and an adversarial approach to generate highly informative test assets and discern the economy's states from numerous macroeconomic series.
➡ Outperforming benchmark models across Sharpe ratio, explained variation, and pricing errors, this model highlights critical factors influencing asset prices, based on data from U.S. stocks (1967-2016) and extensive firm-specific and macroeconomic variables.
➡ With an out-of-sample annual Sharpe ratio of 2.6, it surpasses linear models, deep learning forecasting, and the Fama–French five-factor model in predicting stock returns and explaining their variance.
➡ The model exhibits unparalleled pricing performance across anomaly-sorted portfolios, achieving over 90% R² in cross-sectional analysis, indicating its superior predictive capability and potential impact on asset pricing strategies.
“ChatGPT and Corporate Policies”

ChatGPT is a useful tool for adding new inputs to more complex models, which, in my view, is the best way to utilize it at this point. The paper presents a compelling example of how to leverage ChatGPT to extract and create inputs from conference call transcripts.
Here are my main takeaways from the paper: 👇
➡ The study introduces a firm-level ChatGPT investment score, derived from conference calls, which measures managers' expected changes in capital expenditures.
➡ This score is validated through its strong correlation with CFO survey responses and its ability to predict future capital expenditures up to nine quarters ahead, offering new insights beyond traditional measures like Tobin’s q.
➡ Utilizing data from 74,586 conference call transcripts across 3,878 companies from 2006 to 2020, the research investigates ChatGPT's efficacy in extracting corporate expectations about future investment policies, specifically questioning if ChatGPT can enhance understanding of corporate policies beyond existing indicators.
➡ The investment score not only forecasts total, intangible, and R&D investments but also indicates that firms with high investment scores tend to experience negative future abnormal returns, suggesting the score's potential to influence investment decisions and market perceptions.
➡ This paper demonstrates ChatGPT's broader applicability in evaluating corporate policies such as dividends and employment, highlighting its role in analyzing vast amounts of textual data to provide actionable insights into firms' future investment opportunities and the subsequent effects on asset prices and returns.
➡ The findings underscore the transformative potential of advanced AI models like ChatGPT in financial analysis, offering a more nuanced understanding of corporate investment strategies and their implications for investors, thereby enhancing the decision-making process in the financial domain.
AI-Essentials
I've hand-picked five highly-rated, free machine learning courses to help you enhance your skills. Enjoy! 👇
Macro-Finance Insights
“Macroeconomic forecasting and sovereign risk assessment using deep learning techniques”

Beyond investments, deep learning is becoming a powerful tool for macroeconomic prediction and ultimately supporting monetary policy decisions. This paper serves as an excellent example of this.
Here are my main takeaways from the paper: 👇
➡ This paper presents a cutting-edge method using deep learning to predict the macroeconomic status of countries, focusing on overcoming the limitations of traditional econometric models.
➡ By integrating multiple macro variables, deep learning captures complex, non-linear interdependencies, showcasing superior predictive performance over conventional methods like Bayesian Model Averaging.
➡ The study emphasizes deep learning's versatility in various fields, highlighting its potential in financial forecasting by handling high
dimensional data and recognizing evolving patterns in economic time series.
➡ Demonstrating deep learning's capacity to offer holistic and dynamic forecasts, this approach represents a significant advance in macroeconomic analysis and sovereign risk assessment, providing valuable insights for investment and monetary decisions.
➡ The research aligns computational finance with statistical machine learning, employing deep learning networks to enhance forecast accuracy and reduce errors in economic predictions, setting a new standard for macroeconomic forecasting methodologies.
Ready to elevate your investing journey to new heights?
Unlock the power of informed decision-making with our daily newsletter, tailored to empower investors like you. The Early Bird delivers crucial insights that keep you ahead in the stock market, ensuring you make profitable decisions. Sign up today.
If you're enjoying our newsletter and want to support us, please recommend it to anyone you know who's interested in AI and Finance. Your referrals are the biggest compliment and help us grow! 🌟🤖💼